9 Computational Electromagnetic Imaging of Rough Surfaces
9.1 ROUGH SURFACES FABRICATION BY 3D PRINTING
For both the experimental target fabrication and numerical discretization, digital samples for both Gaussian and exponentially correlated rough surfaces are generated. Unlike the computer generation, it has been difficult to fabricate an exponentially correlated experimental sample by a general-purpose milling machine due to the rich high-frequency roughness. In this regard, the 3D printing technique may be applied for the fabrication. This technique allows one to directly manufacture actual samples based on the computer-generated digital sample with specific parameters, as shown in Table 9.1. More specifically, the FDM (Fused Deposition Modeling) technique is utilized for the fabrication. In this 3D printing process very thin lines of fused materials are continuously printed to construct a structure, and a 100% filling rate can be achieved to form a solid. In this way the shaping accuracy of about 0.5 mm can be achieved, which is approximately λ/20 in the Ka-band and sufficient for the imaging study. The main advantage of this technique is that it permits the usage of lossy material, to generate samples simulating the half-space rough surface scattering scenario, such as the moist soil ground. In this work, the measured permittivity is 6.22-j2.86 at 32 GHz, and that value was used in the computations of the scattering field. Figure 9.1 shows the CAD model and fabricated samples for Gaussian (a) and exponentially (b) correlated rough surface; top panel: CAD model; bottom panel: Fabricated sample. As should be noted, the considered sample has rich high frequency roughness, which generates numerous local scatters, leading to the strong speckle effects.
TABLE 9.1
Parameters of the Rough Surface Samples
Size | RMS Height | Correlation Length | |
Measured Sample | 250 mm in diameter | 4 mm | 48 mm |
(in λ @ 32 GHz) | (26.7 in diameter) | (0.427) | (5.12) |
Numerical Samples | 125 mm × 125 mm | 2 mm, 4 mm, 8 mm, 12 mm | 48 mm |
(in λ @ 32 GHz) | (13.3 × 13.3) | (0.214, 0.427, 0.854, 1.281) | (5.12) |
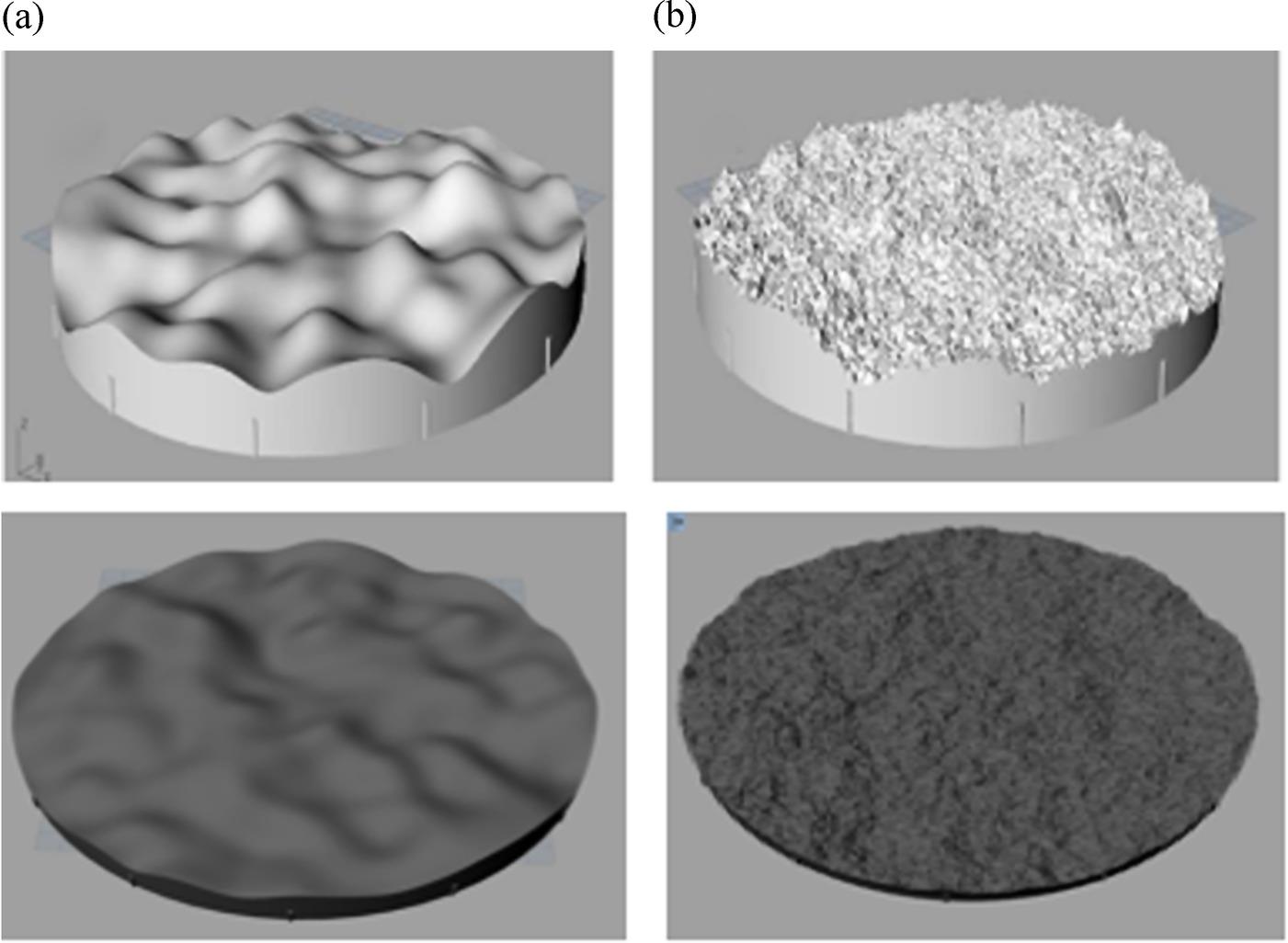
FIGURE 9.1 Geometry Configuration of the 3D model and the fabricated sample of the Gaussian (a) and exponentially (b) correlated rough surface. Top: CAD model; Bottom: Fabricated sample.
To study the speckle properties, we need a large number of computer-generated surface samples. An exponential correlation function for the random rough surface is taken since it describes better the natural ground surface [1]. In this context, the following procedures are excised: first, a very large rough surface mesh is generated on grids, then the digital sample for each realization (400*400 meshing points) is cut from that large mesh. In the cutting, one sample is neighboring and overlapping to next with 100 discretize interval (100*interval size~31 mm) distant, which is close to the footprint size in diameter in the numerical simulations. For the speckle study, 1600 (40*40) samples are obtained for one to achieve an enough number of realizations. The digital samples are then input into the FDTD simulator one by one for computing scattering in the 3D computational domain.
In the context of radar imagery, understanding the speckle properties is imperative for both image de-noising and applications such as land-cover classification and parameter retrieval [1–4]. Radar speckle arises due to the coherent sum of numerous distributed scattering contributions [4]. A general assumption is the “fully developed” speckle model, which is applicable when the resolution cell size is much larger than the correlation length () of the ground surface [4]. This requirement may be met in low-to-medium image resolution. The fully developed speckle model is based on the following two hypotheses: (1) total scattering is contributed by independent scatters; (2) there is a sufficiently large number of scatters contributing to a resolution cell so that a complex Gaussian distribution is followed. For VHR radar images, these assumptions are no longer valid.
Radar backscattering and its speckle statistic variation with the resolution cell size or antenna footprint has been a subject of interest in rough surface scattering [5–9]. In [5], the convergence of backscattering coefficients versus footprint size was studied by 1-D method of moment solution. By theoretical models and indoor SAR experiments in EMSL [6,7], the dependence of polarimetric backscattering characteristics from Gaussian correlated rough surfaces on the SAR resolution cell size was examined, while the SAR images of rough soil surfaces were measured in EMSL [8]. That work includes results and analysis on the backscattering coefficients and image probability density functions (PDF), versus different resolution cell size. As the radar image resolution reaches evolves from tens of meters to approximately one meter, or even a smaller size, this topic becomes more important currently for land observations. To model high-resolution speckle properties, Di Martino et al, proposed the predicting method for the equivalent number of scatterers within the resolution cell [9], considering stochastic stationary rough surface description, and more advanced, fractal surface models. In this work, we address the topic of very high-resolution (VHR) radar speckle statistics using indoor SAR experiments and supporting full-wave simulations.
Since the prediction for the equivalent number of scatterers has been established in [9], it is used as an evaluating tool in this work. To be specific, the exponentially correlated rough surface is considered, due to its rich high-frequency roughness on the surface-air interface that leads to numerous local scattering contributions. In particular, the 3-D printing process was used to fabricate the sample surface that is used for scattering measurement conducted at Ka-band. In this manner, the image resolution scale close to the rough surface correlation length can be achieved, namely, the image resolution cell size can be at the same scale of the surface geometric undulation. In this view, the scattering mechanism from an exponentially correlated rough surface is similar to that from the sea surface, where the scattering process from short scale roughness is modulated by relatively long scale undulation [10,11]. The prediction for the equivalent number of scatterers per resolution cell from [9] is served as a reference in the analysis. Meanwhile, based on the results from experimental and supporting numerical practices considering different incident directions, the effects of scattering scale on the speckle properties can be observed. Further numerical studies are conducted with different RMS height, for discussions on both the factors of the equivalent number of scatterers and the scattering scale effects.
9.2 EXPERIMENTAL MEASUREMENTS AND CALIBRATION
In the scanning measurement, the scattering signals were captured through the antennas and microwave transceiver all along the scanning track and all over the Ka-band in a microwave anechoic chamber as shown in Figure 9.2. Before we proceed with the data acquisition, system calibration is required., as we have already introduced in section 3.42 of Chapter 3. The procedures for polarimetric calibration have been available and well documented in numerous papers [12–16]. We present a general polarimetric calibration technique using hybrid corner reflectors. We deploy only one trihedral and two dihedral corner reflectors with different rotation angles for polarimetric calibration. It is simple and makes no assumptions.
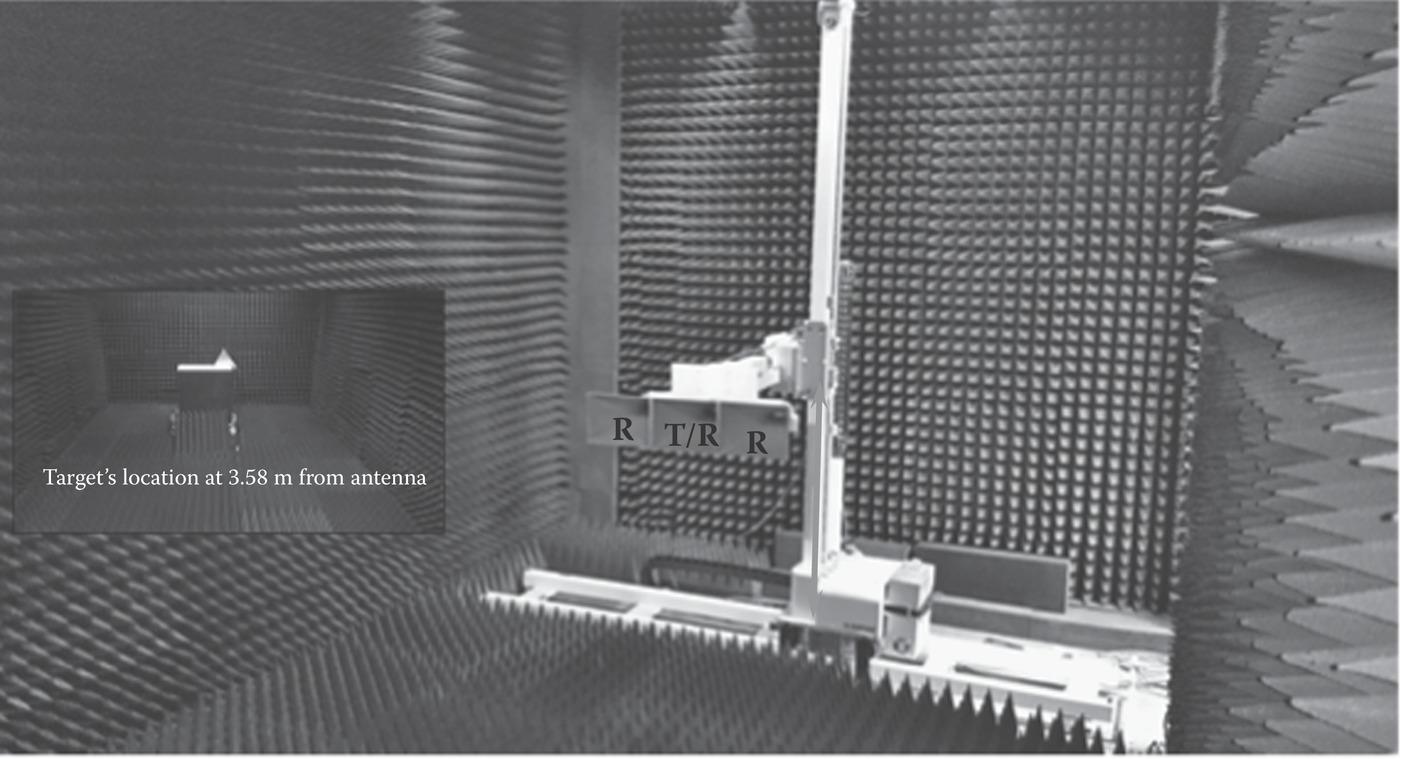
FIGURE 9.2 Measurements at microwave anechoic chamber.
In a real system, under non-ideal conditions, such as polarization cross-talk errors and channel imbalance between transmitter and receiver, the polarimetric calibration is required. This is illustrated in Figure 9.3, where and are the horizontal and vertical polarization components of the incident field, and are the horizontal and vertical polarization components of the scattered field; , , , represent channel imbalance and , , , stand for cross-talk. The wave propagation in transmitting and receiving causes the target scattering matrix distorted to a measured scattering matrix. The goal of the polarimetric calibration is to invert the target scattering matrix from the measured one to obtain a minimum difference. Propagation matrices and noise matrix are acquired from the known and reference targets. We detail the approach to obtaining the matrix elements using the calibration or reference targets as follows.
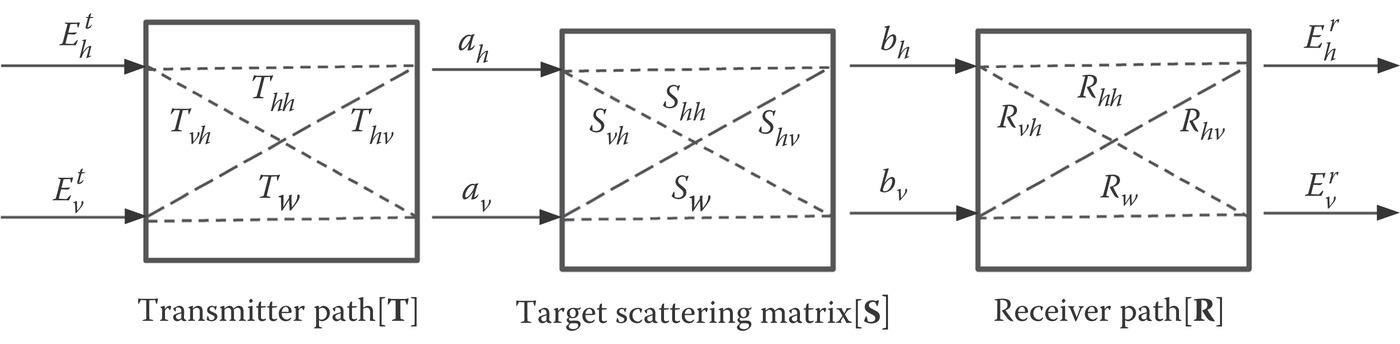
FIGURE 9.3 The process for measuring the target scattering matrix.
Referring to Figure 9.3, in a polarimetric SAR, the measured scattering matrix and true scattering matrix are related by:
where and are the true and measured 2 × 2 scattering matrices, respectively, and represent the transmitting and receiving distortion matrices, respectively, and accounts for random noise. To simplify the numerical calculation, via absorbing all possible elements of and into one matrix without considering the noise contribution involving the scattering reciprocity theorem, Equation 9.1 can be further expressed as follows:
where
The matrix is called the calibration matrix, which contains all distortion in the transmitter and receiver of the polarimetric SAR system. Using eight m matrices in place of the eight matrices, with eight unknowns are given in Equation 9.4:
A total of eight complex unknowns in the calibration matrix need to be solved related to the co-polarizations and cross-polarizations. Therefore, we need at least two calibration targets for co-polarization and one for cross-polarization, forming eight independent complex equations for eight unknowns. In viewing six unknowns for co-polarization, and two unknowns for cross-polarization, passive corner reflectors (CR) are sufficient. They provide more convenient tracking and identification of error sources, if they occur, in the whole wave propagation process. Given these constraints, a trihedral and dihedral corner reflector constitute two equations in co-polarizations, a 45°-rotated dihedral corner reflector makes up two equations in cross-polarization, and a 22.5°-rotated dihedral corner reflector forms four equations in full polarization. Note that the rotation is about the radar incidence plane. The theoretical scattering matrices for the above-selected reflectors are listed in Table 9.2.
TABLE 9.2
Selected Corner Reflectors and Typical Scattering Matrices
![]() |
Based on the theoretical scattering matrices of reference targets, one trihedral and one dihedral are used to solve for the six unknown matrices (, , , , , ), which are connected with co-polarization. The solutions are given in Equation 9.5:
where .. represents the element of the measured scattering matrix of a trihedral corner reflector and similarly denotes the element of measured scattering matrix of a dihedral corner reflector. One 22.5°-rotated dihedral is used to work out the two unknowns (, ), which are related to cross-polarization. The solutions read
where denotes the element of the measured scattering matrix of a 22.5°-rotated corner reflector. After the matrix is determined, the calibrated scattering matrix can be readily obtained from
We employ three reference targets with known measured scattering matrices , and for the polarimetric calibration. We can make use of the relationship between measured and theoretical scattering matrices of reference targets to solve the calibration matrix. After the calibration matrix is determined, the scattering matrices of the reference targets can be calibrated. It follows that the scattering matrices of unknown targets can be calibrated. To assess the accuracy of the proposed calibration technique, the calibrated amplitude and phase error is calculated according to
where represents the calibrated scattering matrices of point targets, and represents the theoretical scattering matrices of point targets.
Another good measure of calibration quality is the calibrator’s polarimetric response, which is a way of visualizing the target scattering properties. We compare the polarimetric responses of the corner reflectors before and after calibration.
9.3 DATA ACQUISITION AND IMAGE FORMATION
To achieve the rough surface imaging in the chamber, the measurements are performed in the Ka–band considering the physical size of the sample prepared in section 9.1. Different bandwidths and synthetic aperture lengths were selected to achieve different spatial resolutions (in ground range), as concluded in Table 9.3. During the image processing, the Kaiser window (β = 2.5) was utilized along with the frequency and aperture signal, and the resolution cell size in Table 9.3 are realized in the presence of the Kaiser window.
TABLE 9.3
Imaging Parameters for Different Resolution Cell Size (Ground Range Resolution, Kaiser Windows ( β = 2.5) Applied in Both Domains)
Ground Resolution | Center Frequency | Aperture Length | Bandwidth (GHz) | |||
30 × 30 mm2 | 32.0 GHz | 240 mm | 11.00 | 8.25 | 6.60 | 6.15 |
45 × 45 mm2 | 160 mm | 7.35 | 5.50 | 4.40 | 4.10 | |
60 × 60 mm2 | 120 mm | 5.50 | 4.13 | 3.30 | 3.07 | |
75 × 75 mm2 | 96 mm | 4.40 | 3.30 | 2.65 | 2.45 | |
90 × 90 mm2 | 80 mm | 3.70 | 2.75 | 2.20 | 2.05 |