The reliability studies depend on a number of indices. These indices are defined in the previous chapters and in the coming chapters (Kim, 2011; Rusin and Wojaczek, 2015). In the present chapter, a number of indices explained. The present chapter highlight on loss of load probability (LOLP), expected un-served energy (EUE), loss of load hours (LOLH), loss of load events (LOLEV), expected power not supplied (EPNS), loss of energy probability (LOEP), energy index of reliability (EIR), interruption duration index (IDI), expected energy not supplied (EENS), energy curtailed, loss of load expectation (LOLE), derated state, and forced outage rate (FOR). The electric power system is the most likely complex system. The system divided into functional areas. These areas called generation, transmission, and distribution. To assess the system reliability, each part to be analyzed separately for easier evaluation and eventually by combining them together. Throughout the period, the resource adequacy metrics explain the occurrence of the risk, which helps the assessment of the power plant. The aggregation of risk across the year of operation might be more suitable to indicate the period of occurrence for resource adequacy risks within the area of operation (IEEE Standard 493–2007). To show the quality and performance of an electrical system, the index LOLP needed. This index value is affected by load growth, the load duration curve, FOR of the plant, number, and capacity of generating units. This index defined as the probability of system as hourly or daily demand exceeding the available generating capacity within a given period. With a daily peak load curve, the LOLP can be calculated. The LOLP is a projected value of how much time the load on a power system is expected to be greater than the capacity of the available generating resources in the end. Therefore, the LOLP is based on combining the probability of generation capacity states with the daily peak probability. Then, as to assess the number of days during the year in which the generation system may be unable to meet the daily peak (Al-Shaalan, 2012; IEEE Standard 493–2007). There are a number of problems that might arise during the use of LOLP of any power system reliability study. These problems are: a. The LOLP does not provide any indication of the duration of shortages or frequency. b. There are different techniques used to find LOLP. These techniques might obtain a different result for the same system. c. The LOLP does not include additional emergency support that one control area may receive from another, even though any region does not include the additional emergency. d. As a result of a future event not modeled by traditional LOLP calculation, major loss of load incidents usually occur. e. The use of the LOLP index would be sufficient for the same system to investigate different expansion plans and an annual maintenance schedule. This is an argue statement and at the same time, it is correct only in case the duration peak load is static over any number of years of the study. f. In the case that the utilities have different shapes of load curve, it is not useful to compare the reliabilities of utilities. g. In most often the cumulative curve of any daily peak load, the used load model, which is used in the loss of load method, is not recognized in that model. h. In the case of building the generation or signing purchasing power system equipment agreement, the LOLP is not important as an accurate predictor of the resulting incidence of electricity shortage. i. The LOLP indicates the number of days in a year that might be lost. This indicates that the generation system is not able to meet the required load during those days. This term belongs to the expected unserved energy. This index measures the expected amount of energy that failed to supply during the period of observation of the system load cycle due to shortages of basic energy supplies. In another way, it can be defined as a measure of the resource availability to serve the required load continuously at delivery points. It can be defined as the expected energy required that will not serve in a given year. The EUE can provide a measure relative to the size of a given assessment area (Billinton and Allan, 1996; IEEE Standard 493–2007). At the same time, the expected number of hours per year can be known as the LOLH when a system’s hourly demand is exceeding the generating capacity. This index can be calculated from the load duration curve. The hourly LOLE is known as LOLH when considering the latest case. The LOLH has a number of classifications known as annual LOLH, monthly LOLH, and annual EUE, which is known as both actual and at the same time, normalized. In a case that some system load is not served during a period of time annually, this case is called LOLEV (NERC, 2016). The event might be an hour or a number of hours. At the same time, this is defined as the number of events where some system load is not served in through the year. A LOLEV can last for one hour or for several number of hours and can involve the loss of one or several hundred megawatts of load. This is known as the frequency of occurrence index (Billinton and Allan, 1996). The EPNS (Elsaiah et al., 2015) is equivalent to the required curtailment since the problem aims to minimize the total load curtailment (Billinton and Allan, 1996). The EPNS is estimated as where: i is the number of cases; LOL is the loss of load. Loss-of-energy probability is a measure for generation reliability assessment (Gold Book, 2007). As a relationship, the LOEP is the ratio of the expected energy not served (EENS) during a certain long period of observation to the total energy demand during the same period (Billinton and Allan, 1996). Using the load duration curve is helping to calculate the LOEP for installed capacity. Therefore, the LOEP is becoming as where the LOEP is unitless and it is a probability; Ei is the energy and it is not supplied due to a capacity outage (Oi); Pi is the probability of the capacity outage (Oi); E during the period of study, and E is the total energy demand. The EIR (Venkatamuni and Reddy, 2014) is given by: where EENS is the expected energy not supplied (Billinton and Allan, 1996). The IDI (Florencias-Oliveros et al., 2018) and (Indiana Utility Regulatory Commission Report) is defined as the IDI (Billinton and Allan, 1996). At the same time, the customer average interruption duration index (CAIDI). The term EENS (or Not Served) is the energy, which is needed to satisfy the load based on the generating units available. In other words, when the load exceeds the available generation, it is called EENS. This means that the energy is reflecting risk. The suitable unit used for the EENS is MWhr per year (Al-Shaalan, 2012; Billinton and Allan, 1996). Example (6.1): Consider the load duration curve shown below. This curve represents a variation of the load duration curve (Figure 6.1) of a small power network. Three units are running to satisfy the above load curve, where the capacities of the units are: Find the EENS for the three periods shown on the curve. Solution: Period #1: To satisfy the first period presented in the curve, the generating-units (2 and 3) are running. Running the second and the third units with their full capacities will keep the network under risk. For this reason, the three units should be running. Period #2: To be in the safe side, the units 1 and 3 can be run together (50MW, 300 MW) Period #3: Units 1 and 3 can be run together (50 MW, 300 MW) If all units (1, 2, and 3) are off, then the EENS will be the total area under the curve. EENS = 1875 + 1500 + 1250 = 4625 MW.hr Unit # Period # 1 2 3 1 OFF ON ON 2 ON OFF OFF 3 ON ON ON
CHAPTER 6
Generating Systems Reliability Indices
6.1 INTRODUCTION
6.2 PROBABILISTIC INDICES
6.2.1 LOSS OF LOAD PROBABILITY (LOLP)
6.2.2 EXPECTED UN-SERVED ENERGY (EUE) AND LOSS OF LOAD HOURS (LOLH)
6.2.3 LOSS OF LOAD EVENTS (LOLEV)
6.2.4 EXPECTED POWER NOT SUPPLIED (EPNS)
6.2.5 LOSS OF ENERGY PROBABILITY (LOEP)
6.2.6 ENERGY INDEX OF RELIABILITY (EIR)
6.2.7 INTERRUPTION DURATION INDEX (IDI)
6.2.8 EXPECTED ENERGY NOT SUPPLIED (EENS)
6.2.9 ENERGY CURTAILED
Due to a shortage in energy where the reason behind is that the demand for electricity exceeds the available power is known as curtailment. Therefore, the term curtailment is one among many tools to maintain system energy balance. It should be known that there are hierarchies of customers. This type of hierarchy is clear where some might require a partial or total cut back of electricity before others. The industrial sectors (industrial customers) are usually curtailed before reducing the service to the residential customers. When electrical energy service has to be interrupted, it means that energy reserves have dropped or are expected to drop below a certain level (Billinton and Allan, 1996; Denholm and Mai, 2019; IEEE Standard 493–2007; Schermeyer et al., 2018).
Example (6.2):
Consider a small electric power system network represented by a load duration curve shown in Figure 6.2. The load curve is represented for a period of 100 hours. The network is running by a generating unit through three-states shown in Table 6.1. Calculate the Energy Curtailed for the considered case. Then, find the generating-unit expected produced energy.
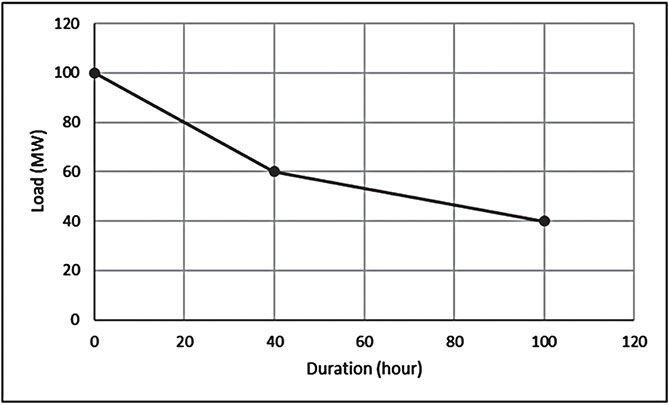
Capacity IN (MW) | Capacity OUT (MW) | Probability |
---|---|---|
50 | 0 | 0.65 |
30 | 10 | 0.30 |
0 | 25 | 0.05 |
Solution:
First, the total energy under the curve is calculated as:
Therefore, the expected energy produce by the unit-generator is the difference between energy under the curve (EENS0, the generating unit or units is/are OFF) and the summation of the expectation of energy curtailed. This means that the generating-unit expected produced energy is 4150 MWhr.
Capacity IN (MW) | Capacity OUT (MW) | Probability | Energy Curtailed (MWhr) | Expectation of E. C. (MWhr) |
---|---|---|---|---|
50 | 0 | 0.65 | 6200–(100×50) = 1200 | 0.65×1200 = 780 |
30 | 10 | 0.30 | 6200–(100×30) = 3200 | 0.30×3200 = 960 |
0 | 25 | 0.05 | 6200–(100×0) = 6200 | 0.05×6200 = 310 |
∑ = 2050 MWhr |
Example (6.3):
Consider a small electric power system network represented by a load duration curve shown in Figure 6.3. The load curve is represented for a period of 100 hours. The network is running by two-generating units through the three-state model and two-state model as shown in Tables 6.2 and 6.3. Calculate the energy curtailed for the considered case. Then, find the generating-units expected produced energy.
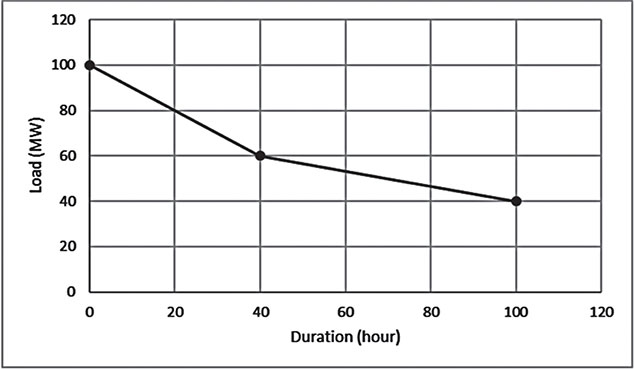
Cap. IN (MW) | Cap. OUT (MW) | Probability |
---|---|---|
25 | 0 | 0.65 |
15 | 10 | 0.3 |
0 | 25 | 0.05 |
Cap. IN (MW) | Cap. OUT (MW) | Probability |
---|---|---|
30 | 0 | 0.97 |
0 | 30 | 0.03 |
Solution:
First, the total energy under the curve is calculated as:
The results are shown in Tables 6.4 and 6.5.
Cap. IN (MW) | Cap. OUT (MW) | Prob. | Curtailed Energy | Expectation of E. C. (MWhr) |
---|---|---|---|---|
25 | 0 | 0.65 | 3700 | 2405 |
15 | 10 | 0.3 | 4700 | 1410 |
0 | 25 | 0.05 | 6200 | 310 |
The total expectation of energy curtailed = 4125 MWhr
Cap. IN (MW) | Cap. OUT (MW) | Prob. | Curtailed Energy (MWhr) | Expectation of E. C. (MWhr) |
---|---|---|---|---|
30 | 0 | 0.97 | 3200 | 3104 |
0 | 30 | 0.03 | 6200 | 186 |
The total expectation of energy curtailed = 3290 MWhr
Joining both generating-units together:
Cap. IN (MW) | Cap. OUT (MW) | Prob. | Energy Curtailed (MWhr) | Expectation (MWhr) | Connect |
---|---|---|---|---|---|
55 | 0 | 0.6305 | 700 | 441.35 | 25 + 30 |
45 | 10 | 0.291 | 1700 | 494.7 | 15 + 30 |
30 | 25 | 0.0485 | 3200 | 155.2 | 0 + 30 |
25 | 30 | 0.0195 | 3700 | 72.15 | 25 + 0 |
15 | 40 | 0.009 | 4700 | 42.3 | 15 + 0 |
0 | 55 | 0.0015 | 6200 | 9.3 | 0 + 0 |
Σ 1 | Σ 1215 MWhr |
6.2.10 LOSS OF LOAD EXPECTATION (LOLE)
The term LOLE is one of the reliability indices. During the observation of the power system load cycle, the expected number of hours (HLOLE: Hourly Loss of Load Expectation) or time period are calculated when insufficient generating capacity is available to serve the required load at a time. In another statement, it can be defined as the expected number of days per annual period in which the available generation capacity is insufficient to satisfy and serve the daily maximum load. It can be calculated from the daily peak load or annual peak load. In practical applications, the LOLE expectation index is more often used than the LOLP probability index. The relationship between both the LOLP and the LOLE is formulated as (IEEE Standard 493–2007):
In case the load model is an annual continuous load curve with day maximum load, then the LOLE unit is in days per year and T = 365 days.
In case the load model is an hourly load curve, then the LOLE unit is in hours per year and T = 8760 hours.
Example (6.4):
A power station is supplying a city in the Kingdom of Bahrain for a year. The weekly average loads have been recorded as such (in MW):
77 | 46 | 41 | 52 |
34 | 41 | 34 | 41 |
41 | 34 | 41 | 57 |
41 | 46 | 57 | 77 |
41 | 52 | 34 | 34 |
57 | 46 | 77 | 41 |
52 | 57 | 41 | 34 |
41 | 41 | 52 | 57 |
34 | 57 | 34 | 52 |
41 | 77 | 34 | 34 |
57 | 52 | 57 | 46 |
77 | 46 | 52 | 34 |
57 | 41 | 52 | 46 |
The power station supplying the city has three generating units, the first unit is working with a capacity of 25MW and 0.02 fail per week. The second unit has a capacity of 25 MW as well, with a failure rate equal to 0.05 f/week. The last unit (number 3) has a repair rate of 0.96 r/week, and its capacity is 50 MW. Calculate the LOLE in percentage.
Solution:
Weekly Average Load (MW) | 77 | 57 | 52 | 46 | 41 | 34 |
No. of Occurrence (week) | 5 | 9 | 8 | 6 | 13 | 11 |
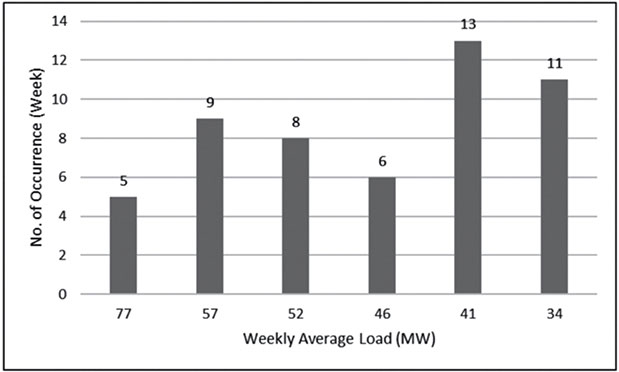
Unit # | Capacity (MW) | Prob. of Success | Prob. of Fail |
---|---|---|---|
1 | 25 | 0.98 | 0.02 |
2 | 25 | 0.95 | 0.05 |
3 | 50 | 0.96 | 0.04 |
|
|
|
|
Capacity IN (MW) | Capacity OUT (MW) | Ind. Probability | Cumulative Prob. |
ON-ON-ON 100 | 0 | (0.98)(0.95)(0.96) = 0.89376 | 0.10624 + 0.89376 = 1.00000 |
ON-OFF-ON OFF-ON-ON 75 | 25 | (0.98)(0.05)(0.96)+ (0.02)(0.95)(0.96) = 0.06528 | 0.04096 + 0.06528 = 0.10624 |
OFF-OFF-ON ON-ON-OFF 50 | 50 | (0.02)(0.05)(0.96)+ (0.98) (0.95)(0.04) = 0.0382 | 0.00276 + 0.0382 = 0.04096 |
ON-OFF-OFF OFF-ON-OFF 25 | 75 | (0.98)(0.05)(0.04)+ (0.02) (0.95)(0.04) = 0.00272 | 0.00004 + 0.00272 = 0.00276 |
OFF-OFF-OFF 0 | 100 | (0.02)(0.05)(0.04) = 0.00004 | 0.00004 |
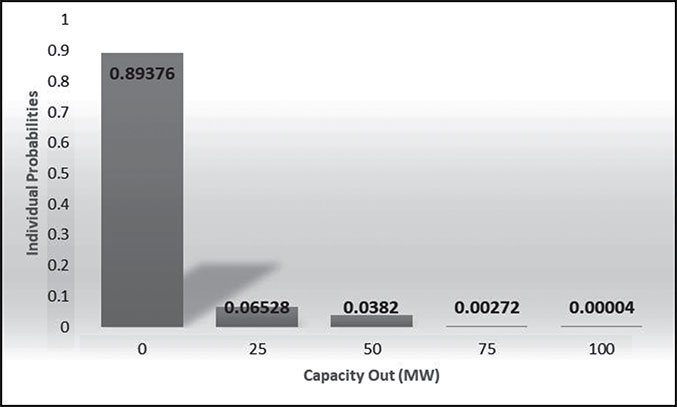
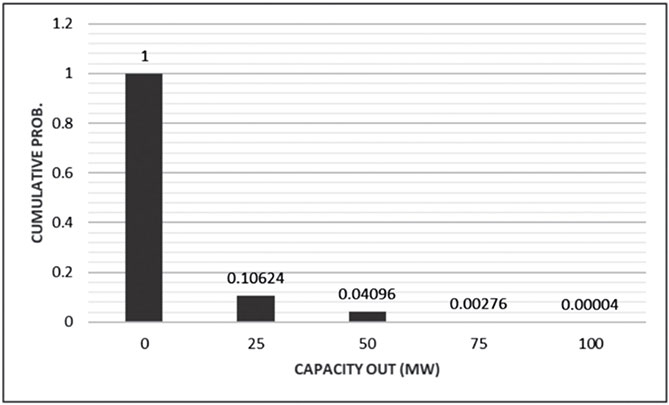
where: the maximum available power is the maximum capacity.
The expected loss of load through the year is approximately equal to 1.3 week. This is equivalent to 9.17 days a year.
Example (6.5):
A power station is supplying a city in the Kingdom of Bahrain for a year. The weekly average loads have been recorded as such (in MW):
68 | 92 | 82 | 104 |
82 | 114 | 82 | 114 |
92 | 82 | 154 | 68 |
114 | 154 | 114 | 68 |
82 | 114 | 68 | 114 |
82 | 68 | 154 | 104 |
68 | 82 | 114 | 82 |
104 | 82 | 154 | 114 |
114 | 104 | 82 | 92 |
68 | 82 | 104 | 82 |
82 | 68 | 154 | 104 |
92 | 104 | 68 | 92 |
68 | 104 | 92 | 68 |
The power station supplying the city has three generating units, the first unit is working with a capacity of 50MW and 0.02 fail per week. The second unit has a capacity of 50MW as well, with a failure rate equal to 0.05 f/week. The third unit has a repair rate of 0.96 r/week, and its capacity is 100MW. Calculate the LOLE in percentage.
Solution:
Weekly Average Load (MW) | 68 | 82 | 92 | 104 | 114 | 154 |
No. of Occurrence (week) | 11 | 13 | 6 | 8 | 9 | 5 |
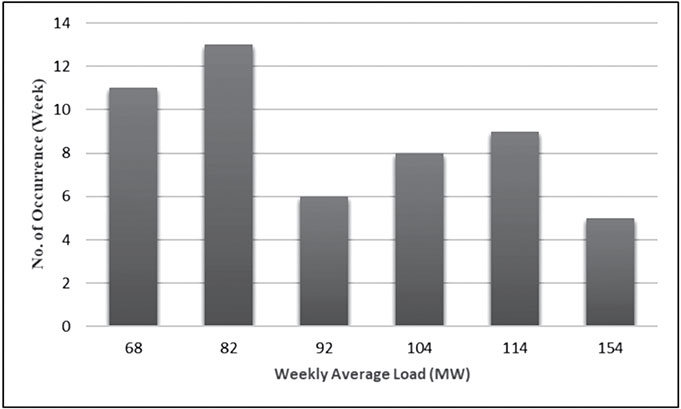
Unit # | Capacity (MW) | Probability of Success | Probability of Fail |
---|---|---|---|
1 | 50 | 0.98 | 0.02 |
2 | 50 | 0.95 | 0.05 |
3 | 100 | 0.96 | 0.04 |
|
|
|
|
Capacity IN (MW) | Capacity OUT (MW) | Individual Probability | Cumulative Probability |
ON-ON-ON 200 | 0 | (0.98)(0.95)(0.96) = 0.89376 | 0.10624 + 0.89376 = 1.00000 |
ON-OFF-ON OFF-ON-ON 150 | 50 | (0.98)(0.05)(0.96)+ (0.02) (0.95)(0.96) = 0.06528 | 0.04096 + 0.06528 = 0.10624 |
OFF-OFF-ON ON-ON-OFF 100 | 100 | (0.02)(0.05)(0.96)+ (0.98) (0.95)(0.04) = 0.0382 | 0.00276 + 0.0382 = 0.04096 |
ON-OFF-OFF OFF-ON-OFF 50 | 150 | (0.98)(0.05)(0.04)+ (0.02) (0.95)(0.04) = 0.00272 | 0.00004 + 0.00272 = 0.00276 |
OFF-OFF-OFF 0 | 200 | (0.02)(0.05)(0.04) = 0.00004 | 0.00004 |
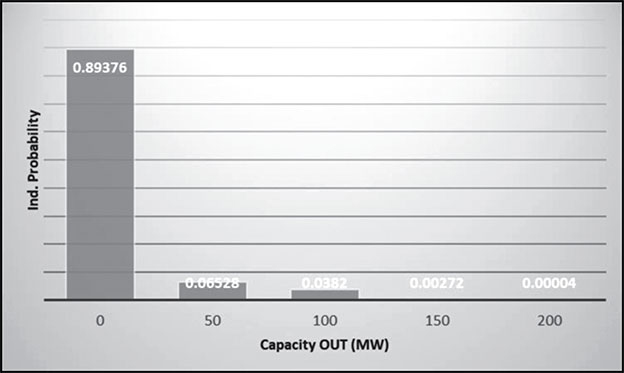
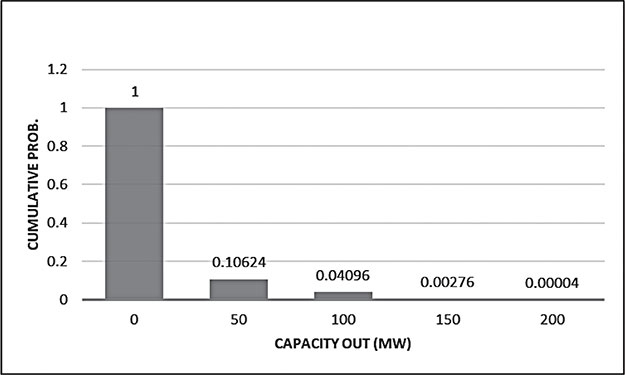
where: the maximum available power is the maximum capacity.
The expected loss of load through the year is approximately equal to 1.3 week. This is equivalent to 9.17 days a year.
Example (6.6):
Three generators have been running for 52 weeks. These generators are represented by the following data summarized in the following table:
Unit # | Capacity (MW) | Prob. of Success | Prob. of Fail |
---|---|---|---|
1 | 50 | 0.998 | 0.002 |
2 | 60 | 0.995 | 0.005 |
3 | 100 | 0.992 | 0.008 |
The table below shows the data of the peak loads it occurred and how frequent it is happening:
Weekly Average Load (MW) | 68 | 82 | 92 | 104 | 114 | 154 |
No. of Occurrence (Week) | 11 | 13 | 6 | 8 | 9 | 5 |
Calculate the LOLE in percentage.
Solution:
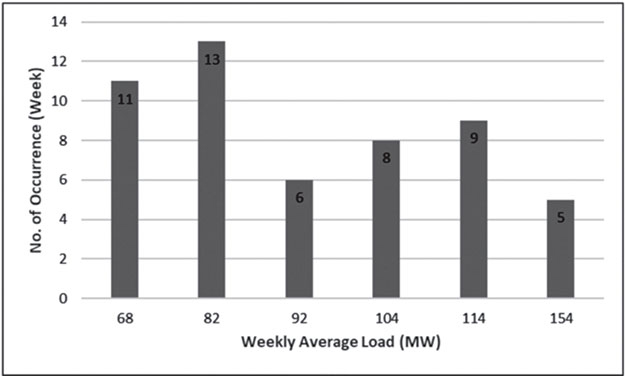
Capacity IN (MW) | Capacity OUT (MW) | Ind. Probability | Cumulative Prob. |
---|---|---|---|
ON-ON-ON 210 | 0 | (0.998)(0.995)(0.992) = 0.98506592 | 1.00000000 |
OFF-ON-ON 160 | 50 | (0.002)(0.995)(0.992) = 0.00197408 | 0.01493408 |
ON-OFF-ON 150 | 60 | (0.998)(0.005)(0.992) = 0.00495008 | 0.01296 |
ON-ON-OFF 110 | 100 | (0.998)(0.995)(0.008) = 0.00794408 | 0.00800992 |
OFF-OFF-ON 100 | 110 | (0.002)(0.005)(0.992) = 0.00000992 | 0.00006584 |
OFF-ON-OFF 60 | 150 | (0.002)(0.995)(0.008) = 0.00001592 | 0.00005592 |
ON-OFF-OFF 50 | 160 | (0.998)(0.005)(0.008) = 0.00004 | 0.00004 |
OFF-OFF-OFF 0 | 210 | (0.002)(0.005)(0.008) = 80 × 10−9 | 80 × 10−9 |
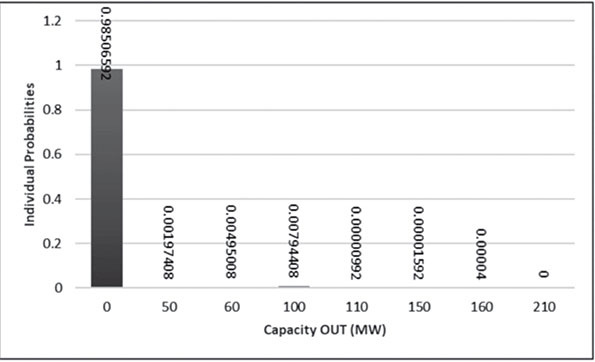
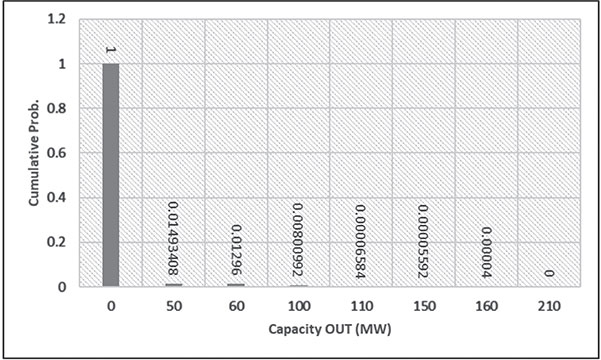
where: the maximum available power is the maximum capacity.
The expected loss of load through the year is approximately equal to 2.77 weeks. This is equivalent to 19.4 days a year.
Example (6.7):
Three generators are running with the following data:
Unit # | Capacity (MW) | λ (f/day) | μ(r/day) |
---|---|---|---|
1 | 25 | 0.02 | 0.98 |
2 | 25 | 0.03 | 0.97 |
3 | 50 | 0.04 | 0.96 |
The three generators have been running for one year (365 days). The data for a period of one year with a maximum of 100MW are given in the following table:
Daily Peak Load (MW) | 57 | 52 | 46 | 41 | 34 |
No. of Occurrence (days) | 12 | 83 | 107 | 116 | 47 |
Find the LOLE.
Solution:
Unit # | Probability of Success | Probability of Fail |
---|---|---|
1 | 0.98 | 0.02 |
2 | 0.97 | 0.03 |
3 | 0.96 | 0.04 |
To combine the three units together as one system:
Capacity In (MW) | Capacity Out (MW) | Individual Probability | Cumulative Probability |
---|---|---|---|
ON ON ON 100 | 0 | (0.98)(0.97)(0.96) = 0.912576 | 0.912576 + 0.087424 = 1.000000 |
ON OFF ON OFF ON ON 75 | 25 | (0.98)(0.03)(0.96)+(0.02)(0.97) (0.96) = 0.046848 | 0.046848 + 0.040576 = 0.087424 |
ON ON OFF OFF OFF ON 50 | 50 | (0.98)(0.97)(0.04)+(0.02)(0.03) (0.96) = 0.038600 | 0.038600 + 0.001976 = 0.040576 |
ON OFF OFF OFF IFF ON 25 | 75 | (0.98)(0.03)(0.04)+(0.02)(0.97)(0.04) = 0.019520 | 0.019520 + 0.000024 = 0.001976 |
OFF OFF OFF 0 | 100 | (0.02)(0.03)(0.04) = 0.000024 | 0.000024 |
where: the maximum available power is the maximum capacity.
Daily Peak Load (MW) | 57 | 52 | 46 | 41 | 34 |
No. of Occurrence (days) | 12 | 83 | 107 | 116 | 47 |
This figure shows that the system might loss power for 4.38824 days in a year. This means that the expected number of hours per year that the system’s electricity production cannot meet its demand.
The loss of load percentage = (4.38824 / 365) × 100 = 1.2327%
6.2.11 DERATED STATE
Usually, the term derating is used in the electric power systems and electronic devices. At the same time, the derating as a term used when a component rated less than the maximum capability of it. In the field of electric power systems, the expected excess of available generation capacity over electric power demand is known as the derated capacity margin (Billinton and Allan, 1992; IEEE Standard 493–2007). The de-rated capacity margin statement is used; because uncontinuous plant generation depending on weather conditions, and at the same time, the plants provide uncertain levels of generation capacity. At a case of some generating-units at non-peak demand times are under maintenance, the system could be considered under the derated state. As an example, the three-state model is considered as shown in Figure 6.4. This model has the following states:
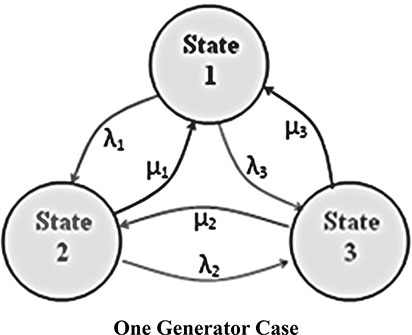
• State (1): it means that the system is operated with full capacity.
• State (2): it means that the system is working with the partial of its capacity (which is defined as a derated-state).
• State (3): it means that the system is in the fail state.
Therefore,
6.2.12 FORCED OUTAGE RATE (FOR)
This index varies between the resource types and areas. The FOR can be also defined in a formula shape as (Billinton and Allan, 1992; IEEE Standard 493–2007):
6.2.13 AVAILABILITY AND UNAVAILABILITY
The availability (Davidov and Pantos, 2019) of the system has a number of formulas:
At the same time, when the system has two-states the availability of the system is given by
In case, the system has three states: up-state, derated-state, and downstate. Therefore,
This means the availability is considered that the system is under operation. The unavailability means that the system is in the fail state.
6.3 GENERATION SYSTEM RELIABILITY EVALUATION (MEASUREMENT)
Any power system is evaluated based on a number of points, these points are summarized as (Akhavein and Porkar, 2017; Al-Shaalan, 2012; Chaiamarit and Nuchprayoon, 2013; Zhong et al., 2017):
a. The unit(s) availability during the operation time.
b. The unit(s) unavailability during the operation time.
c. The system components, which are classified as repairable or non-repairable.
d. The components might be in service or out of service.
e. The state probabilities need to known.
f. The load forecast during the electric network operation.
6.3.1 GENERATION AND LOAD MODELS
The generation model (Ma et al., 2018; Staveley-O’Carroll, 2019) is represented in its simplest form, which is a two-state model. This means that it will pass through the up-state and down-state. This case has been discussed earlier. The load model (Wang et al., 2018; Lv et al., 2018; Zheng et al., 2019) is represented by the load curve.
6.3.2 SYSTEM RISK INDICES
A combination of the generating capacity and the load models will result in the system risk indices. A random sequence of N number of load levels is known as a load cycle for the period (Mosaad et al., 2018; Yu et al., 2018). The N number of load periods must be an integer. As an example, if a daily load model has a peak load level of mean duration e day (e is known as the exposure factor) and a fixed low load of (1 – e) day. The electric load exposure factor is represented in the impact of the risk over the load asset, or percentage of load asset lost. As an example, if the load asset value is reduced two thirds, the load exposure factor value is 0.667. If the asset is completely lost, the exposure factor is unity. Therefore, this case can be represented as a daily load model curve as illustrated in Figure 6.5.
With reference to Figure 6.5, the value of X is proportional inversely to the electric load exposure factor (e). This means that when the value of (X) decreases, (e) increases. The electric load cycle can be presented as shown in Figure 6.6.
The parameters needed to completely define the load cycle period shown in Figure 6.6 are summarized as shown in Table 6.6.