8 Radar Imaging Techniques
8.1 STOCHASTIC WAVE EQUATIONS
Radar as a coherent imager is physically based on the wave equations that govern the behavior of wave-targets interactions through the process of radiation, transmission, propagation, and reflection/refraction. For a randomly rough surface being imaged, the governing waver equations become stochastic [1], as we already considered in Chapter 5. Here, we briefly introduce the stochastic wave equations at a minimum but sufficient level to serve as fundamentals of radar imaging.
Consider the radar imaging of a nonmagnetic random medium as shown in Figure 8.1. The locations of the transmitter Tx and receiver Rx are in bistatic mode. For more the general random medium, it is specified by the constitute relations as given in 3.2 of Chapter 3.
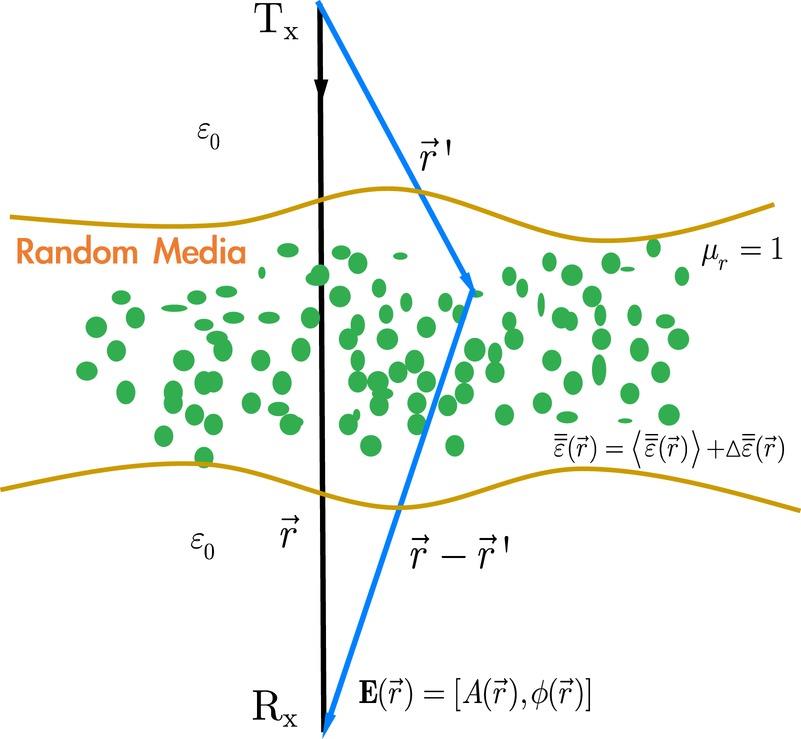
FIGURE 8.1 Radar imaging of a random medium.
Similarly to the problem we deal with the Huygen’s principle, the total field E received at Rx is the sum of the transmitted field Et at Tx and scattered field Es from the random medium under the presence of the incident field, as already introduced in Equation 1.18:
where is tensor Green’s function; k0 is the free space wavenumber. is objection function, and may be any other forms. The random medium is described by permittivity , where is the mean permittivity and is the fluctuating permittivity, with . In the absence of the random medium, the objection function , the total field equals the transmitted field. Noted that depending on the location of the receiver, the transmitted field may propagate through the medium. But it would not affect the problem solving, which is by way of applying the boundary conditions.
The scattered field appearing in Equation 8.1 is given rise by scattered by random scatters, scattering from random rough surface, or fluctuating propagation in random continua inside the random medium intercepted by the transmitting antenna and receiving antenna. Because of the random properties of the permittivity, the total field is composed of a mean-field and a fluctuating field [1–3]. In radar, it is commonly named as a coherent and inherent field, correspondingly, similar to our treatment of scattering field in Chapters 4 and 5. Theoretically, decomposing of the total field into a coherent field and incoherent field is mathematically straightforward. In practice, it is not so as the antenna patterns always exercise influence, making it is difficult to differentiate the coherent returns from incoherent returns in total returns. In transmitting the signal toward the medium, an unmodulated waveform is rarely used. Broadly, there are two basic types of waveform: pulse and continuous wave. As such, in microwave remote sensing, linear frequency modulated (LFM) pulse (chirp), frequency modulated continuous wave (FMCW), and step frequency modulated (SFM), are among commonly used waveforms. Proper choice of waveform determines the radar capability of resolving range and azimuth ambiguities and spatial resolutions, and of course depends on the application scenario. It also involves the radar sensitivity, measurement precision, and dynamic ranges [4].
Equation 8.1 constitutes a stochastic vector wave equation to determine the received field. The problem risen here is how to solve Equation 8.1. This is indeed a computational electromagnetic problem. Many excellent books are available to offer solutions for various kinds of boundary values problems [5–9]. A thorough treatment of such problems is not possible and is beyond the scope of this book. Readers can always consult the source of these books to seek a preferred approach. Indeed, with the advances of both software programing (e.g., massively parallel) and hardware development (e.g., graphic computation unit), the computational electromagnetic problem is still in its accelerating progress toward offering higher computationally efficient and accurate solutions as never before. For random medium imaging, an analytical solution to Equation 8.1 is rarely feasible. Two types of numerical solutions are usually devised: full-wave solution and approximate solution. In a full-wave solution, the problem can be treated in time domain or frequency domain, or hybrid. The method of moment (MoM) in the frequency domain and Finite difference time domain (FDTD) is well-known. The full wave approach implies that the solution contains all bounces of scattering, between radar-targets, and among targets. Imaging formation based on full wave solution therefore poses much lesser information loss in terms of preserving the complete scattering process.
Major advances have been made in numerical solutions of Maxwell equations in three-dimensional simulations (NMM3D) [10,11] for rough surface scattering. As an application example, with the fast and yet accurate numerical simulation method at hand, it is not difficult to study the effects of the surface inhomogeneity. By viewing the Green’s function of layered inhomogeneous media, it is noted that the reflect term that accounts for the inhomogeneous effects is needed in addition to a direct term. Mathematically, this is simple and straightforward. Numerically, it is not really so, however. After some mathematical manipulations, it is possible to split the reflect term and cast them into the original algorithm with minimum modifications. we describe the governing equations and the calculation of incident fields and scattered fields of a dielectric rough surface with a finite extent. In numerical solutions of Equation 8.1, only a surface of finite area can be considered. An area of Lx by Ly is discretized. A finite surface will cause edge diffraction of the incident wave. However, considering the practical case of an antenna radiation pattern that usually falls off, or making so, to the edge of the surface, the diffraction may be ignorable. We chose to apply MoM to solve Maxwell equations and the surface electric fields and magnetic fields are calculated. These surface waves are the “final” surface fields on the surface and include the multiple scattering of the monochromatic tapered wave within the surface area of Lx by Ly. The electric fields of the scattered waves are calculated by using Huygen’s principle and are obtained by integration of the “final” surface fields, weighted by the Green’s functions, over the area of Lx by Ly. The simulation of the scattering matrix is the calculation of scattered fields for two incident polarizations, vertical and horizontal polarizations. The two incident waves and h are of the same frequency and incident on the same realization of a rough surface. The scattering matrix elements include all the multiple scattering within the area Lx by Ly. The scattering matrix elements are normalized by the square root of the incident power as they are calculated from the scattered electric field.
Consider a plane wave, and , with a time dependence of , impinging upon a two-dimensional dielectric rough surface with a random height of . The incident fields can be expressed in terms of the spectrum of the incident wave [10,11]
For horizontally polarized (TE) wave incidence
and for vertically polarized (TM) wave incidence
with and . The incident wave vector is , and denote the polarization vectors. In the above k and η are the wavenumber and wave impedance of free space, respectively. In practical cases, the incident field is tapered so that the illuminated rough surface can be confined to the surface size . The spectrum of the incident wave, , is given as
where and
The parameter βe, a fraction of surface length L, controls the tapering of the incident wave such that the edge diffraction is negligible. Physically, βe is similar to finite beamwidth of the antenna. The tapered wave is close to a plane wave if βe is much larger than a wavelength. The tapered incident wave still obeys, as should be, Maxwell equation because only the wave-vector spectrum of the incident wave is modified from that of a plane wave. With the incident waves defined above, now the surface fields satisfy equations:
where S′ denotes the rough surface, a source point, and a field point on the rough surface. The unit normal vector refers to primed coordinate and points away from the second medium.
The dyadic Green’s functions of free space, , is
where is unit dyadic. The dyadic Green’s function of the transmitted medium, an inhomogeneous layer, , consists of two parts: a direct part and a reflected part:
The direct part is the same as the one for homogeneous medium
which can be put into a vector form and the reflected part that accounts for the layered effects is given as [5,7]
where
The reflection coefficients for horizontal and vertical polarizations, Rh and Rv, respectively, are readily obtained through the recurrence relation assuming that the general inhomogeneous layer is represented by N layers of piecewise constant regions. Please refer Chapter 3 for details. This formulation is applicable if the first layered medium interface is below the lowest point of the rough surface , i.e., . To evaluate Equation 8.19, a double infinite integral is required to compute. The numerical approach proposed in [12] is applied in this paper. As a result, a total of nine elements of and a total of eight elements of are numerically evaluated. These elements represent the contributions from the inhomogeneous layers to the total scattering. The reformulation is given in the next section, while the complete components for numerical computation are given in Appendix 8B. When the lower medium is homogeneous, vanishes, and the problem reduces to those homogeneous rough surface scattering. We then show that how the reflected part of the dyadic Green’s function is cast into formulation such that the modification of the numerical coding can be minimized within the framework of the physics-based two-grid method (PBTG) [12,13]. Equations 8.12–8.14 are written in the matrix equations using moment of method with pulse function as basis function and point matching method
where are unknown surface fields needed to be solved, and are given by the incident fields, and the parameter N is the number of points we use to sample the rough surface. For which correspond the surface integral equation when approaching the surface from free space and for when approaching the surface from the lower medium. The quantities of are zero for .
where
are surface unknowns and the slope tilting factor is , and are surface slopes along x and y directions, respectively.
The in Equation 8.23 is the impedance elements and are determined by the free-space Green’s function and the lower medium Green’s function. The parameter N is the number of points we use to digitize the rough surface.
To solve Equation 8.23, we obtain the surface fields. Traditionally, the matrix equation is solved by matrix inversion or Gaussian elimination methods, which requires O(N3) operations and O(N2) memory. To keep the structure of the PBTG algorithm as much as possible, the surface fields associated with the reflected part of the dyadic Green’s function is decomposed into tangential and normal components for and fields. For inhomogeneous surfaces, there are additional tangential fields, associated with the , and normal fields, associated with need to be solved. In the following, we illustrate our derivation for the reflected part of the Green’s function. The final results can be put into the structure of PBTG and thus the fast method can be applied.
Consider the integral equation of the form
Taking the tangential projection, we reach the following forms
Following the notations of PBTG, Equations 8.32 and 8.33 can be rewritten as
What we need is to write up explicit forms of and . This is given in Appendix 8A. Now that are new terms resulting from and are readily added on the original six scalar integral equations for the homogeneous medium. The rewritten makes the inclusion of the inhomogeneous effects without difficulties by simply casting them into the numerical computation framework. The inclusions of these terms in the generation of impedance matrix slightly increase the computation time. Numerically calculations involving and are illustrated below.
As the formulations made in the above section, the inclusion of the reflected part of the dyadic Green’s function that accounts for the inhomogeneity of the lower medium under the framework PBTG method is straightforward. In formulating the impedance matrix and thus in the calculation of the matrix elements, additional efforts must be exercised to compute the matrix elements with double infinite integral involving and . In this aspect, we adopted the method proposed by Tsang et al. [12]. The method evaluates the matrix elements by numerically integrating the Sommerfeld integrals along the Sommerfeld with higher-order asymptotic extraction. Written in spectral form, is given by
and the curl of Equation 8.36 is
More explicit forms of Equations 8.36 and 8.37 are given in Appendix 8B. Following the numerical procedures proposed in [12], they are calculated in high numerical stability and accuracy. Finally, the reflection coefficients Rv, Rh are given for completeness [2].
where or polarization, and dl represents region depth in region l.
We have insofar presented a full-wave solution of Equation (8.1) by the technique of Galerkin method, in particular the PBTG-based MoM for an inhomogeneous rough surface, as an illustrated example. Another approach to solving Equation (8.1) is an approximate solution. Since Equation (8.1) is Fredholm’s integral equation of the second kind [14], an iterative approach is suitable, and perhaps, is the most appropriate to seek an approximate solution T.
The solution to a general Fredholm integral equation of the second kind is called an integral equation Neumann series. By iteration, we can account for the order solutions to which we are satisfied with specific problems at hand. In view of the iterative approach to solving Equation (8.1), if we start with the initial guess for the unknown field inside the integral using the incident field, we have the following total field as
Equation (8.39) states for the known transmitted field, the total field, and hence the scattered field is determined immediately by carrying out the integration over the space covering the random medium within the reach of antenna footprint. Such a solution is the first-order solution to account for the single scattering process and is known as the first-order Born approximation [15]. To make the first-order Born approximation applicable, the scattered field must be much weaker than the incident field:
For our object function of interest in Equation (8.1), condition of Equation (8.40) imposes that
To be more strictly, we not only require the dielectric contrast against the background that supports the object must be small, but the electrical size of the object also matters, where the size means the maximum extent subtended by the antenna footprint, as illustrated in Figure 3.11. Hence, if the object is electrically larger, the range of dielectric contrast of Equation 8.41 becomes even smaller.
If we keep on the iterative process until reaching convergence, we obtain the Neumann series. So the Born approximation the first term of Neumann series.
Another commonly used approximation is Rytov approximation [15], which was supposed to improve the predictions by the geometric optics and Born approximation. For the first-order solution, Rytov approximation reduces to geometric optics solution if the diffraction scattering is ignored, and is found to reduce to Born approximation if the scattered field amplitude and phase fluctuations are both small. In this context, it has been argued that Rytov approximation and Born approximation have the same domain of validity [16,17]. The advantages of one over the other approximation still count on the degree of media inhomogeneity and the wavefields. The basic Rytov approximation is to replace the unknow field inside the integral of Equation 8.1 using an exponential form:
Then expanding into power series in the fluctuated part of the permittivity in random media. Noted that in Born approximation, the series expansion does not converge when the phase fluctuation is greater than unity, both first-order Born approximation and Rytov approximation remain still linear integral equations to the object function . Hence, the accuracy of the Rytov approximation is more sensitive to the phase variations due to the dielectric contrast. However, unlike Born approximation, the Rytov approximation releases the limitation of the electrical size of the object. For dealing with wave scattering from the rough surface or random media, Born approximation is usually preferred. On the other hand, if we concern with the wave propagation in the media, namely, dealing with the transmitted filed, then Rytov approximation gives a more accurate solution.
Another popular approximate solution to the wave equation is the parabolic equation (PE) approximation [18,19]. Instead of treating it exhaustively, we simply brief it. For those interested readers, an excellent book can be referred [18]. The basic assumptions to make PE work fine include that most of the energy propagating is confined to the so-called paraxial direction, and the dielectric contrast against the surrounding background is small and smooth. Whether we take the full-wave solution or approximate solution, once the surface fields are solved, the scattered fields at the far-zone can be computed according to the Stratton and Chu formula given in Equations 4.9–4.10 of Chapter 4.
8.2 TIME-REVERSAL IMAGING
As briefly introduced in Chapter 1, it is of great practical significance to image the targets obscured by complex random media using the time-reversal (TR) technique [15,20–24], which is essentially a spatiotemporal matched filter to focus the target image adaptively. However, a space-space multi-static data matrix TR is somewhat difficult to achieve both high resolution under highly noisy interference. A modified time-reversal imaging method, formed by space-frequency multi-static data matrix, or space-frequency time-reversal multiple signal classification (TR-MUSIC) may be preferred. In Chapter 1, we have illustrated that the TR-MUSIC can offer a high capability of imaging targets in the presence of a random medium.
The space-frequency TR-MUSIC imaging utilizes the full backscattered data, including the contributions of all multiple sub-matrices, and is found to be statistically stable. Using the backscattered data collected by an antenna array, a space-frequency multi-static data matrix (SF-MDM) is possibly configured. Then the singular value decomposition is applied to the matrix to obtain the noisy subspace vector, which is then employed to image the target. Based on the statistical modeling of random media, the space-space TR-MUSIC and space-frequency TR-MUSIC imaging of the target obscured by random media are compared and analyzed. Numerical simulations show that the imaging performance of the space-frequency TR-MUSIC is better than that of the traditional space-space TR-MUSIC in both free space and random media.
Referring to Figure 1.6, the time-reversal array, consisting of N antenna units, is considered an N-input and an N-output of the linear time-invariant system. Let hlm(t) be the impulse response between the antenna array element m and the antenna array element l, including all propagation effects of the random medium and system noise between the array elements. Assume the input signal is , and the output signal is given by
where denotes the convolution over time. The Fourier transform of rl(t) is
In matrix notation, Equation (8.44) is expressed by
where R(w) and E(w) are transmitting and receiving signals vector in the frequency domain, respectively, H(w) is the transmission matrix, whose dimension is the number of antennas in time-reversal array. By duality in Green function, the transmission matrix H(w) is symmetric, namely, for all matrix elements l, m, .
Assuming the nth element of TR array transmits a Gaussian beam of the form as given in Equation 1.37 or the time-domain of the form:
where T is pulse duration; , fc is carrier frequency, and all the N elements of the TR array receive the scattering signal and take M sampling points, then the SF-MDM for the received signal in the frequency domain, Kn(w), can be written as:
where is the signal bandwidth. The singular value decomposition of gives
where Un is the order matrix representing the left singular vector, Vn is the order matrix representing the right singular vector, and is the singular value matrix of order . When there are targets, there exist singular values greater than zero, corresponding singular vectors forming the signal subspace, and the remaining singular vectors singular values near zero is regarded as the noise subspace. The target information is embedded in the amplitude and phase of the singular vector of the signal subspace the target. The time-reversed signal that needs to be reversed is:
where is spectrum form of s(t). It follows that the TR-MUSIC imaging function is given by
which is based on a single space-frequency matrix, and the steering vector is
with being the background Green’s function.
We see that the decomposed left singular vector forms an orthogonal set containing sensor position information, while the right singular vector forms an orthogonal set containing frequency information. It turns out that Kn(w) represents a space-frequency multi-static data matrix. The decomposition of Kn(w) is called space-frequency decomposition, and the imaging based on such space-frequency decomposition is called space-frequency TR-MUSIC.
Recalled that Equation (8.51) is the data matrix for the nth element of the TR array to transmit and the rest of the elements to receive the scattering signal. Now, if every element from 1 to N sequentially transmits the signal, while the rest of the elements receive simultaneously the scattering signal, then the full data matrix of becomes
with
The singular value decomposition of K(w) is
where U is the left singular vector matrix of order , Λ is the singular value matrix of order , V is the right singular vector matrix of order. It follows that the time-reversal signal is
and imaging function for the full data matrix is of the form
The above matrix is TR-MUSIC based on full data from the elements of the TRE array in playing the signal transmission and reception. We may interpret Equation 8.50 as a single-input multiple-output (SIMO) system, while Equation (8.56) is a multiple-input multiple-output (MIMO) system. A quick comparison of SIMO and MIMO performance for imaging targets in free-space is shown in Figure 8.2. A noise level of 0 dB is added. Both SIMO and MIMO offer better azimuth resolution than range resolution. The SIMO space-frequency TR-MUSIC imaging presents a range shifts, while MIMO space-frequency TR-MUSIC imaging seems to have not such range displacement.
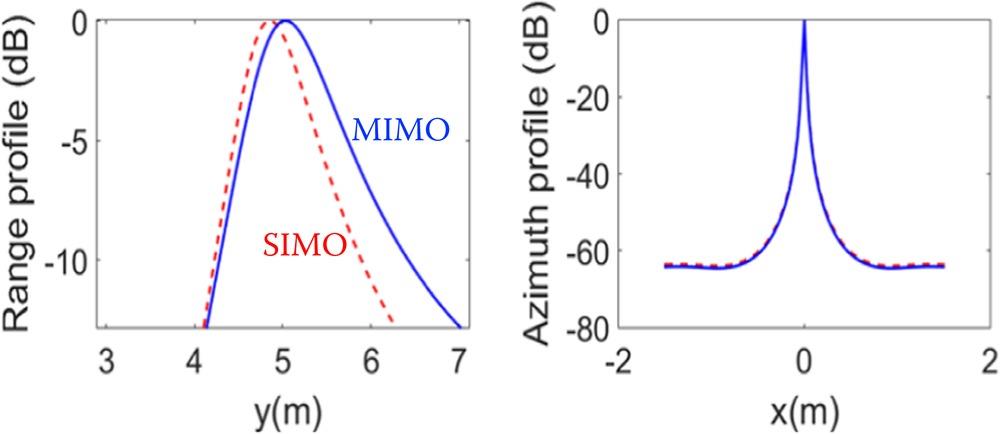
FIGURE 8.2 Comparison of space-frequency TR-MUSIC imaging target in free-space by SIMO and MIMO: range profile and azimuth profile. The noise level was set to 0 dB.
For the imaging of a target in a random medium background, assuming that the target is an isotropic scatterer with a constant scattering amplitude, with the imaging scene shown in Figure 1.6. For N elements of TR array, we have a transmission matrix:
where is the ensemble average; is the scattering coefficient, T denotes the matrix transpose, and
The matrix element of K is the transmission function between element i and element j given by a random Green’s function as:
Then the Decomposition of the Time Reversal Operator (DORT) T is
where denotes the conjugate transpose operator, and the elements of T are
For the strong and weak fluctuations of the wave propagation in a random media, the circular complex Gaussian approximation imposes constraints. Assuming the circular complex Gaussian distribution, the fourth-order moment appearing in Equation (8.61) can be approximated from the second-order moment [25]:
The second order moment is recognized as a mutual coherence function:
Using the parabolic equation (PE) approximation, we have
where Gio is the free-space Green’s function:
where is 0-order Hankle function of first kind. The factor accouts for random medium contribution. One possible solution is given in [26].
Now, the K matrix in full data (MIMO) space-frequency TR-MUSIC writes
and
where the elements are
Following the same procedure in obtaining the imaging function of Equation 8.52 by singular value decomposition applying to Equation 8.67, we can readily perform TR-MUSIC imaging for target obscured by random medium. Table 8.1 gives the simulation parameters to compare the performance between the space-space and space-frequency TR-MUSIC. For imageing geometry, please refer to Figure 1.6.
TABLE 8.1
Simulation Parameters Setting of Space–Space and Space–Frequency TR-MUSIC Imaging
Parameters | Symbol | Value |
Pulse duration | T | 4 ns |
Carrier frequency | fc | 3 GHz |
Number of elements | N | 11 |
Element spacing | 0.05 m | |
Pixel spacing | 0.025×0.025 m2 | |
d1 | 1.2 m | |
Medium depth | d2 | 1.3 m |
d3 | 2.5 m | |
Target location | T1 | T1(0 m, 5 m) |
Albedo | 0.1/0.5/1 | |
Optical depth | OD | 0.1/1/5 |