7 Selected Model Applications to Remote Sensing
An analytical scattering model presented in Chapter 4 has been validated by comparison with numerical simulations and measurements from ground-based, airborne, and spaceborne sensors. The sensitivity test on the surface parameters response to radar return was presented in Chapter 5. In the previous chapter, issues regarding the parameter estimation from radar measurements have been discussed. The mapping from parameter domain to data (or image) domain, and vice versa, is a highly nonlinear problem. Various techniques for information retrieval from remotely sensed data have been proposed in many recent studies—they are too numerous to cite all of them here. Some of them are based on an empirical relationship between the measured return signals and the ground truth. Because they developed from a limited number of observations, these models are generally valid only for the conditions under which those measured data were taken. For example, some of these models also appear that no dependence on the roughness parameter, correlation length. In this chapter, we presented a few model application examples: soil moisture retrieval and directional finding of the incident source.
If one perceives radar remote sensing—a stochastic electromagnetic wave scattering problem—more closely and deeply, the following the 4Vs characteristics may be recognized: volume, variety, velocity, and veracity. As pointed out by Hey et al. [1], the data science in the big data era combines and synergizes the observation, model prediction, and numerical simulation such that data transforms into information, and knowledge can be assured. Within a context of radar imaging, as we see from the 4Vs, more advanced data analytics apparently should be developed to explore richer information offered by fully bistatic radars with fully polarized remote sensing data, which is the main objective of this paper. Hence, it can be realized that a better solution of inverting rough surface parameters is that, by knowing the scattering patterns, one may be able to detect the presence of undesired random roughness of a reflective surface (e.g., an antenna reflector), and thus accordingly, and perhaps effectively, devise a means to correct or compensate phase errors. In many aspects, parameters retrieval from radar measurements is a highly nonlinear problem, yet an important objective in radar remote sensing of surfaces. The advance of neural network and deep learning [2–4] has provided an effective and yet efficient approach to retrieving these surface parameters.
7.1 SURFACE PARAMETER RESPONSE TO RADAR OBSERVATIONS—A QUICK LOOK
To get started, we present the AIEM backscattering predictions with experimental measurements from ground-based, airborne, and spaceborne sensors. Sample data from POLARSCAT Data [5], EMSL data [6], and SMOSREX06 Data [7] are used to illustrate the radar response to the surface parameters, though more details have been presented in previous chapters. These data were summarized and discussed in [8].
7.1.1 COMPARISON WITH POLARSCAT DATA
Remember that in Chapter 4, we illustrated a model prediction comparing with these data sets, but in a manner of correlation plot. The correlation plots of backscattering coefficients between the AIEM and the measured data for HH, VV, and HV polarizations are shown in Figures 4.12 and 4.13. Figure 4.12 shows the data for three frequency bands, and Figure 4.13 shows the data by separating the wet and dry conditions. Here, we would like to expand the comparison into more detail [8].
7.1.1.1 For Surface 1 (S1)
A total of three different soil surfaces with exponential autocorrelation function measured at three different frequencies (1.5, 4.75, and 9.5 GHz) ranging from 20° to 50° were used. The comparisons of AIEM predictions with the POLARSCAT measurements under different soil surfaces. The backscattering coefficients simulated by AIEM for an exponentially correlated surface with σ = 0.4 cm, = 8.4 cm at L/C/X-band under dry and wet soil conditions, are shown in Figures 7.1 and 7.2, respectively. It is seen visually that the angular trends of AIEM predictions generally coincide with the POLARSCAT data at all three frequencies. As expected, the backscattering coefficient decreases with increasing incident angle and increases with decreasing frequency from a smooth surface. Besides, it can be observed that the AIEM predictions agree better with the POLARSCAT data at smaller incident angles (e.g., 20° and 30°) while slightly deviate at lager incident angle (e.g., 40° and 50°), especially for HH polarization.
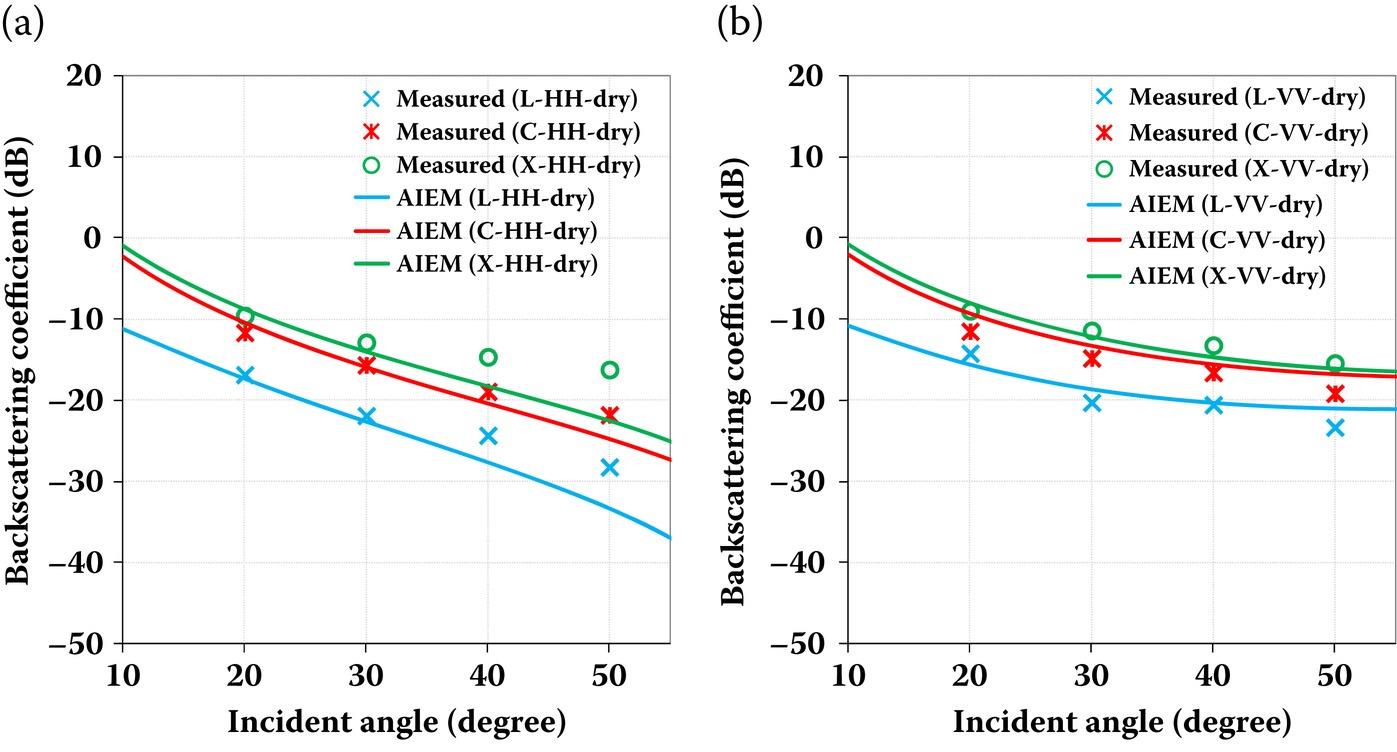
FIGURE 7.1 Comparison of backscattering coefficient between AIEM and POLARSCAT data for an exponential correlated surface with σ = 0.4 cm, = 8.4 cm at 1.5 GHz ( = 7.99-j2.02), 4.75 GHz ( = 8.77-j1.04) and 9.5 GHz ( = 5.70-j1.32) for (a) HH polarization and (b) VV polarization.
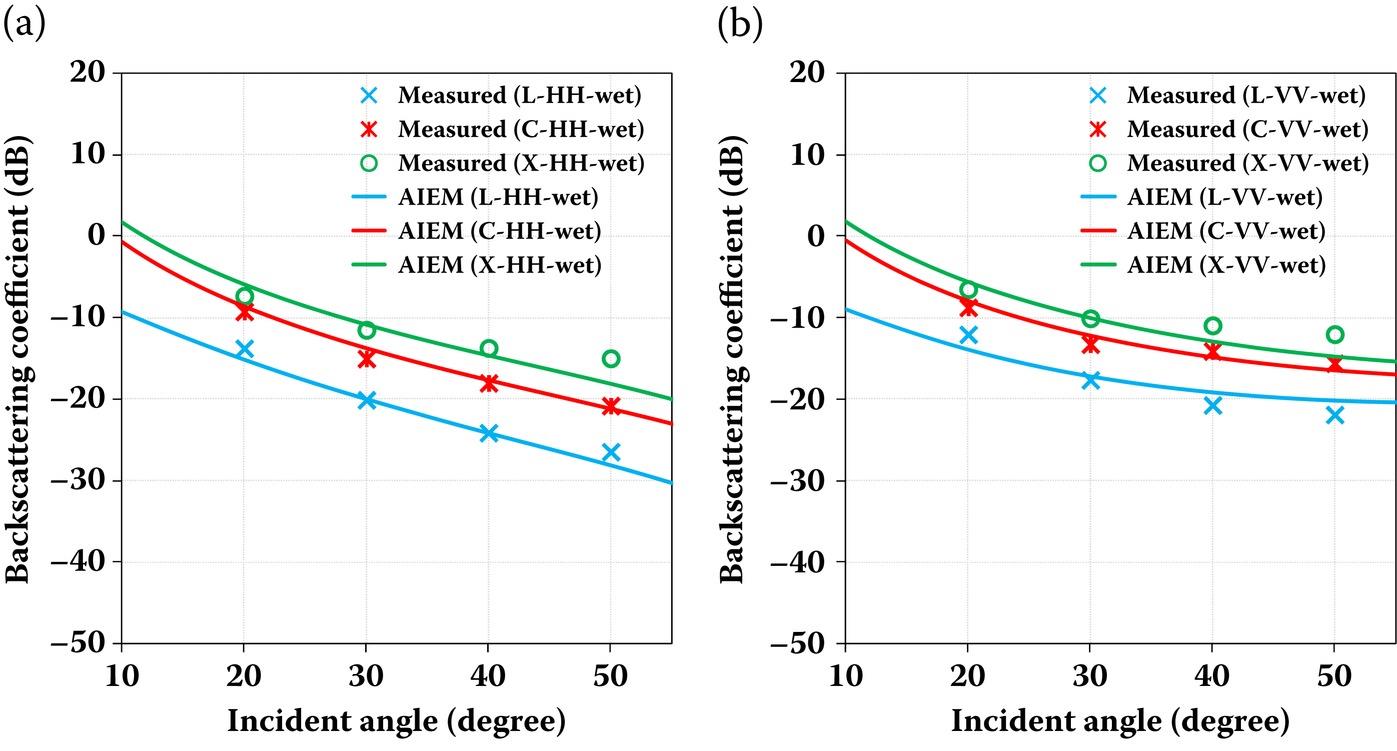
FIGURE 7.2 Comparison of backscattering coefficient between AIEM and POLARSCAT data for an exponential correlated surface with σ = 0.4 cm, = 8.4 cm at 1.5 GHz ( = 15.57-j3.71), 4.75 GHz ( = 15.42-j2.15) and 9.5 GHz ( = 12.31-j3.55) for (a) HH polarization and (b) VV polarization.
7.1.1.2 For Surface 2 (S2)
We compare the model predictions with the measurement data for the second soil surface (i.e., S2), and the results are shown in Figures 7.3 and 7.4. In general, the AIEM predictions are in good agreement with the POLARSCAT measurements over the most of the angular range under consideration, but deviates for HH polarization for incident angle larger than 40° under the dry soil conditions. It can be observed that the experimental measurements do not decrease monotonically with the increase of incident angle particularly for the L-band under dry soil conditions. This perhaps was attributed from measurement noises. As observed from the results of surface 1, the AIEM performs much better under wet soil conditions than under dry conditions for surface 2. This may be explained by the gradually increase of volume scattering in the dry soil surfaces that is somehow difficult to characterize [9].
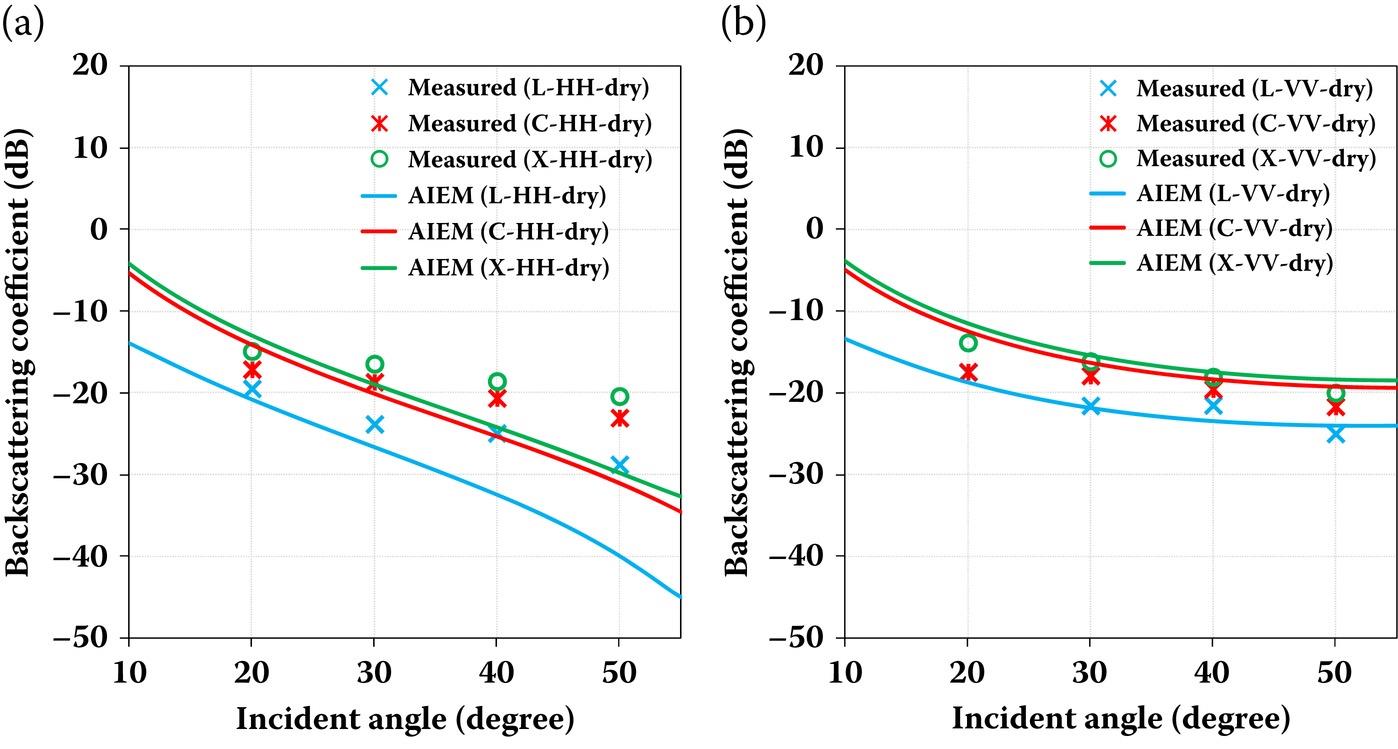
FIGURE 7.3 Comparison of backscattering coefficient between AIEM and POLARSCAT data for an exponential correlated surface with σ = 0.32 cm, = 9.9 cm at 1.5 GHz ( = 5.85-j1.46), 4.75 GHz ( = 6.66-j0.68) and 9.5 GHz ( = 4.26-j0.76) for (a) HH polarization and (b) VV polarization.
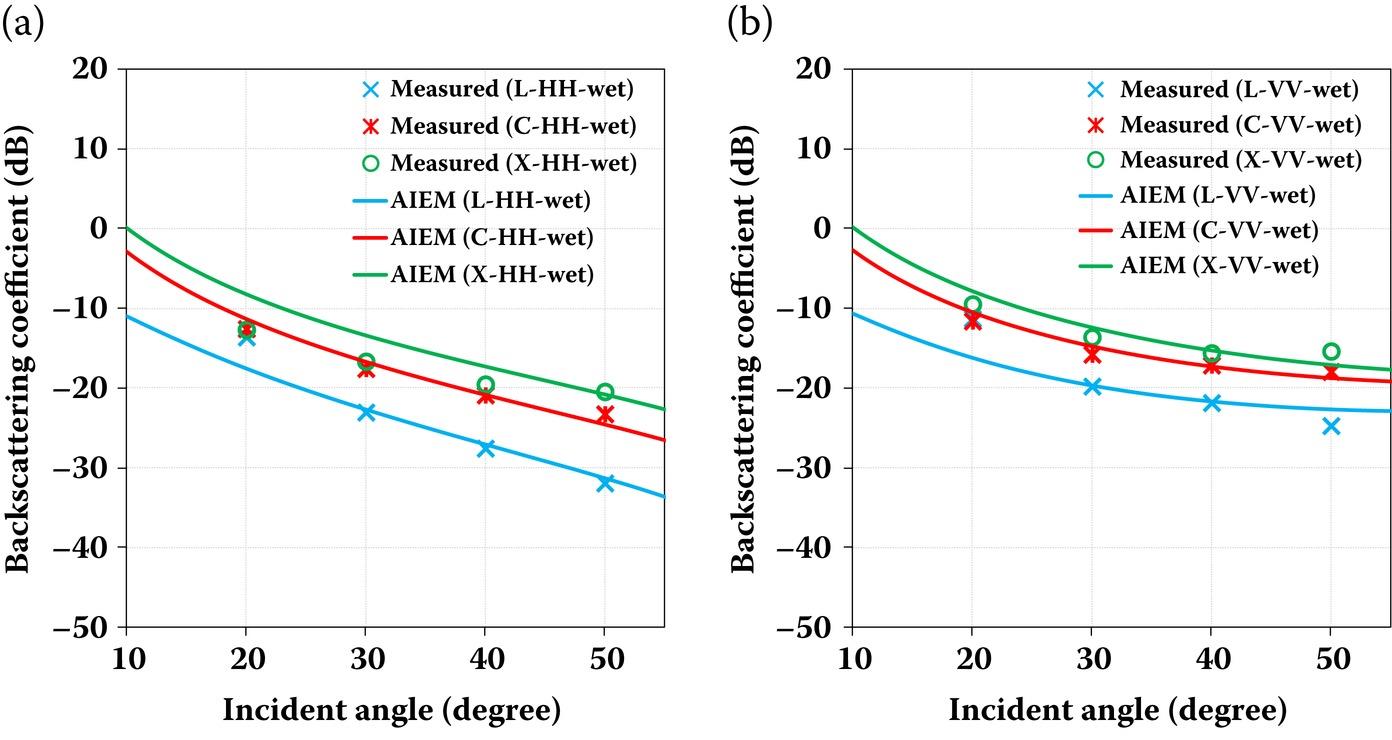
FIGURE 7.4 Comparison of backscattering coefficient between AIEM and POLARSCAT data for an exponential correlated surface with σ = 0.32 cm, = 9.9 cm at 1.5 GHz ( = 14.43-j3.47), 4.75 GHz ( = 14.47-j1.99) and 9.5 GHz ( = 12.64-j3.69) for (a) HH polarization and (b) VV polarization.
7.1.1.3 For Surface 3 (S3)
In what follows, we continue to assess the accuracy of AIEM backscattering simulations with the POLARSCAT data, shown in Figures 7.5 and 7.6. In this case, the RMS height is 1.12 cm, and the correlation length is 8.4 cm, which corresponds to the roughest surface among the three experiments. Due to the increased roughness, the scattering curve over all angles becomes flat. Again, the AIEM predictions well capture the angular trends of the POLARSCAT data at all frequencies for both HH and VV polarizations. It is also observed that in this case, the measurements at C-band and X-band are nearly the same regardless of the incident angle, whereas there is an obvious separation between the HH and VV polarized backscattering coefficient predicted by the AIEM over the incident angles. Insofar, we only illustrate the co-polarization. For discussion on cross-polarized backscattering, please refer to Chapter 4, and reference [10] for details.
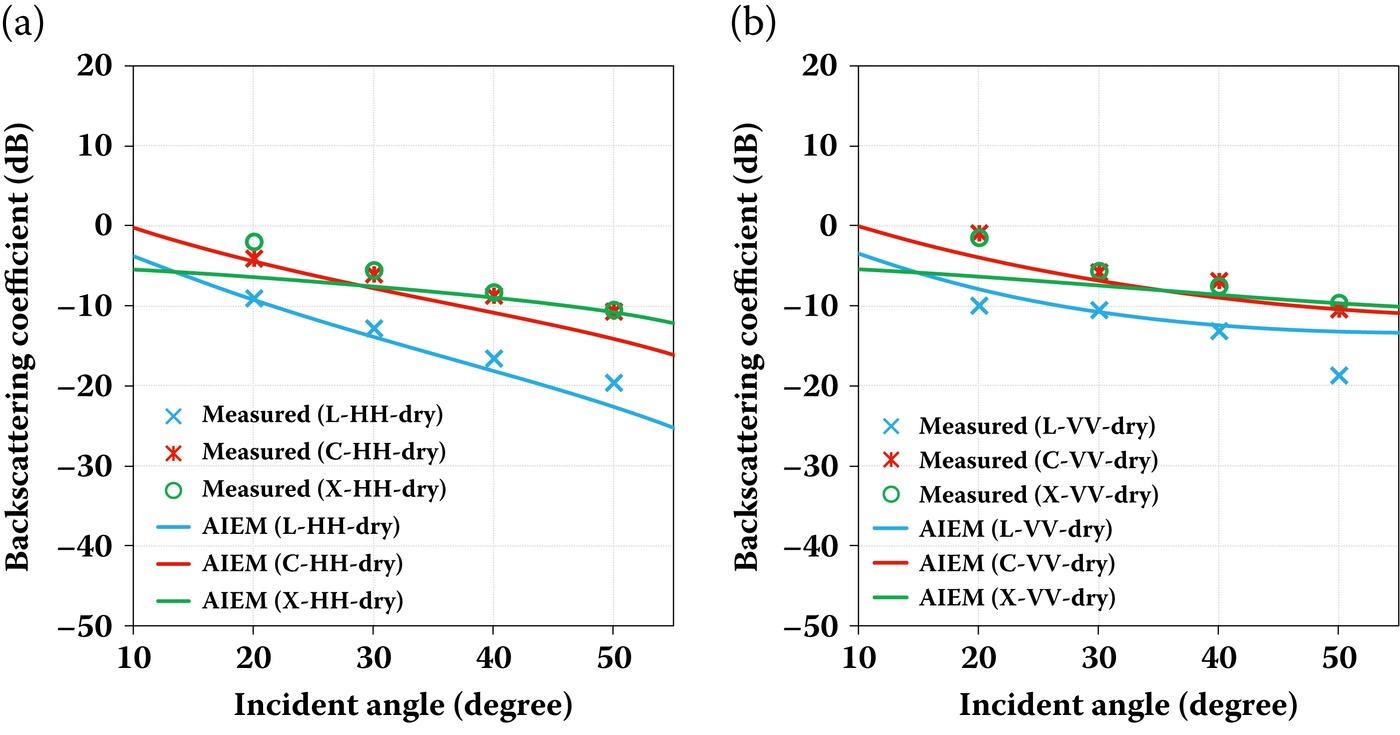
FIGURE 7.5 Comparison of backscattering coefficient between AIEM and POLARSCAT data for an exponential correlated surface with σ = 1.12 cm, = 8.4 cm at 1.5 GHz ( = 7.70-j1.95), 4.75 GHz ( = 8.50-j1.00) and 9.5 GHz ( = 6.07-j1.46) for (a) HH polarization and (b) VV polarization.
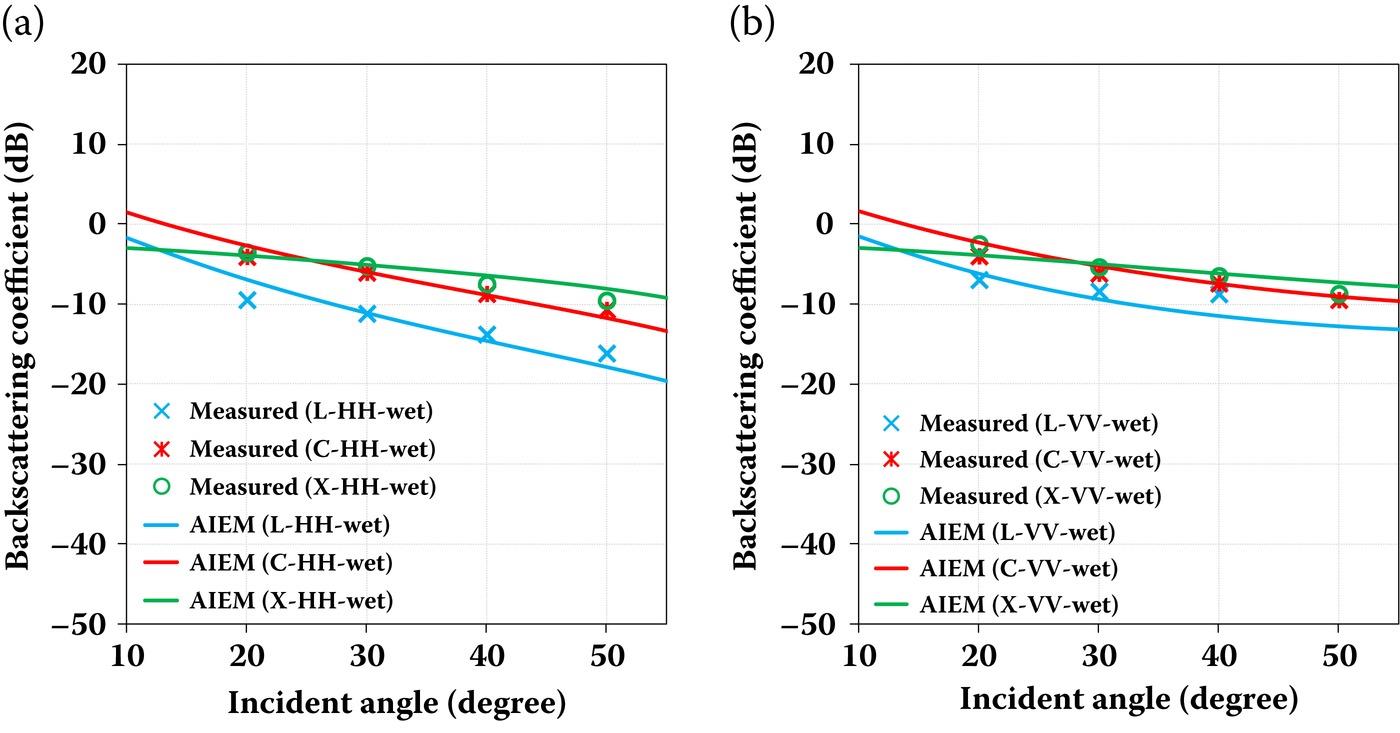
FIGURE 7.6 Comparison of backscattering coefficient between AIEM and POLARSCAT data for an exponential correlated surface with σ = 1.12 cm, = 8.4 cm at 1.5 GHz ( = 15.34-j3.66), 4.75 GHz ( = 15.23-j2.12) and 9.5 GHz ( = 13.14-j3.85) for (a) HH polarization and (b) VV polarization.
7.1.2 COMPARISON WITH EMSL DATA
The bistatic scattering measurement data used here was acquired by the EMSL [6]. The EMSL data were performed on a Gaussian correlated surface with σ = 0.4 cm, = 6 cm, and incident angle of 20° and scattering angle between 0° and 50° at two frequencies (i.e., 11 and 13 GHz). The comparison between the AIEM predictions and the EMSL measurements is shown in Figure 7.7. It can be observed that the bistatic scattering behavior of AIEM is generally in agreement with the EMSL data. The flatness of the angular trend between (10° and 30°), a forward scattering region, in measurements seems unexplainable, because in these scattering angles, a stronger scattering signal is physically expected. Nevertheless, the AIEM well predicts the bistatic scattering measurements for both HH and VV polarizations with a favorable RMSE value within 2.5 dB and a correlation value greater than 0.96.
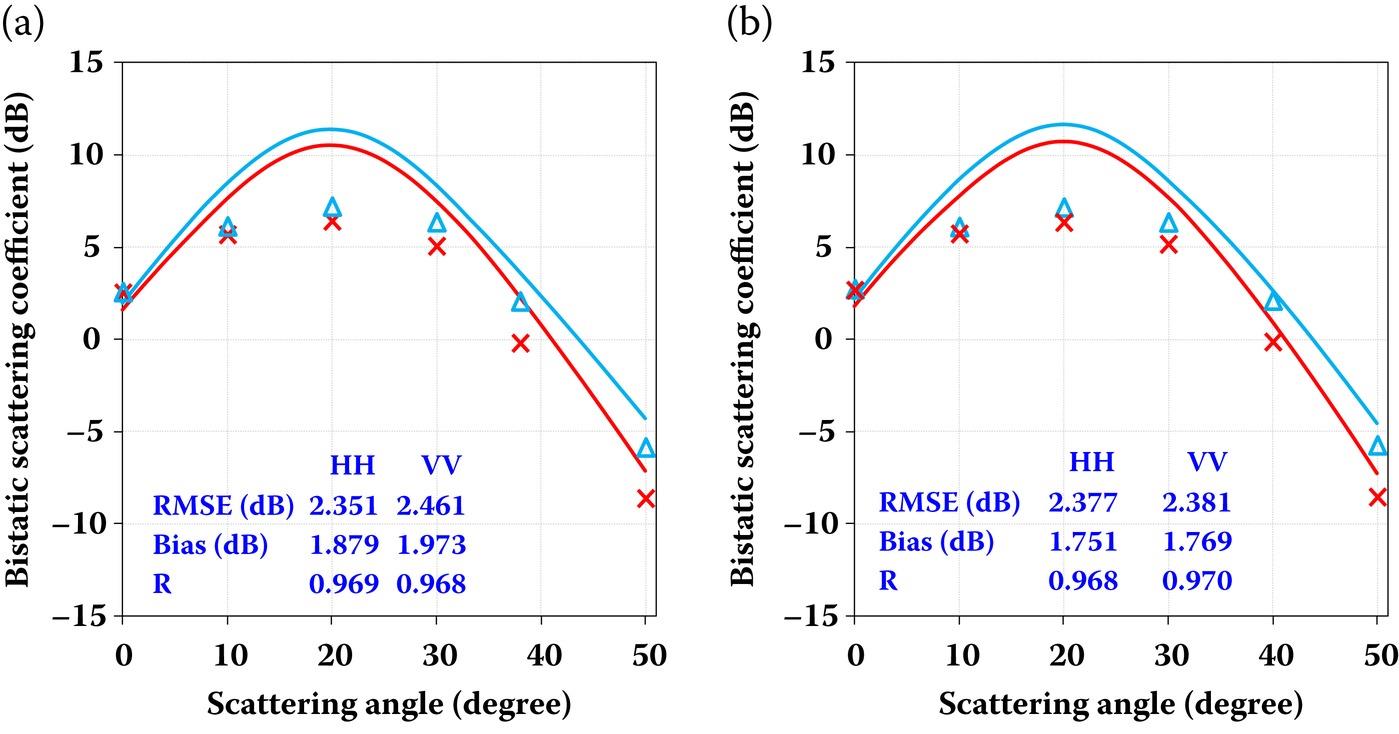
FIGURE 7.7 Comparison of bistatic scattering coefficient between AIEM and EMSL measurements for a Gaussian correlated surface with = 5.5-j2.1, σ = 0.4 cm, = 6 cm, and incident angle of 20° for (a) 11 GHz and (b) 13 GHz.
7.1.3 COMPARISON WITH SMOSREX06 DATA
For a rough surface, the noncoherent reflectivity is obtained by integrating the bistatic scattering coefficient over the upper hemisphere. Because of the reciprocity, the emissivity is the same as absorptivity, the amount of power absorbed by the dielectric in a scattering problem. Hence, it would be useful to examine the model prediction of the surface emissivity. Also, in this regard, the active and passive microwave remote sensing is interplayed.
The comparisons of the emissivity predicted by AIEM and the SMOSREX06 measurements are illustrated in Figure 7.8. Here, the performances of AIEM are observed in terms of angular and polarization dependences. It is seen that, in general, the AIEM predictions are fairly close to the measured emissivity at small to intermediate look angles, from 20° to 40°, for both H and V polarizations. However, when the look angle increases to 50° and 60°, the gap between these two data becomes larger, especially for H polarization. It is found that both the H and V polarized emissivities simulated by AIEM underestimate the SMOSREX06 measurements. Increasing the look angle, the dry bias at H polarization also increases, which is not observed at V polarization, however. Indeed, the RMSE is not quite satisfactory, particularly at large look angles (e.g., 50° and 60°) for H polarization. The AIEM predictions correlate well with the measured emissivity with the correlation coefficient close to 0.9 for both H and V polarizations. In Figure 7.8, we see a fairly well linear relationship between the AIEM predictions and measurements. Since the observation period of the measurements is very long, collecting a total of 8125 samples and the comparison, as presented here, is made without adjusting the given input parameters to the AIEM model, it is convinced that the AIEM is able to correctly predict the temporal dynamic of the observed surface emissivity over a wide range of look angle and surface parameters. More on the error measures are referred to [8].
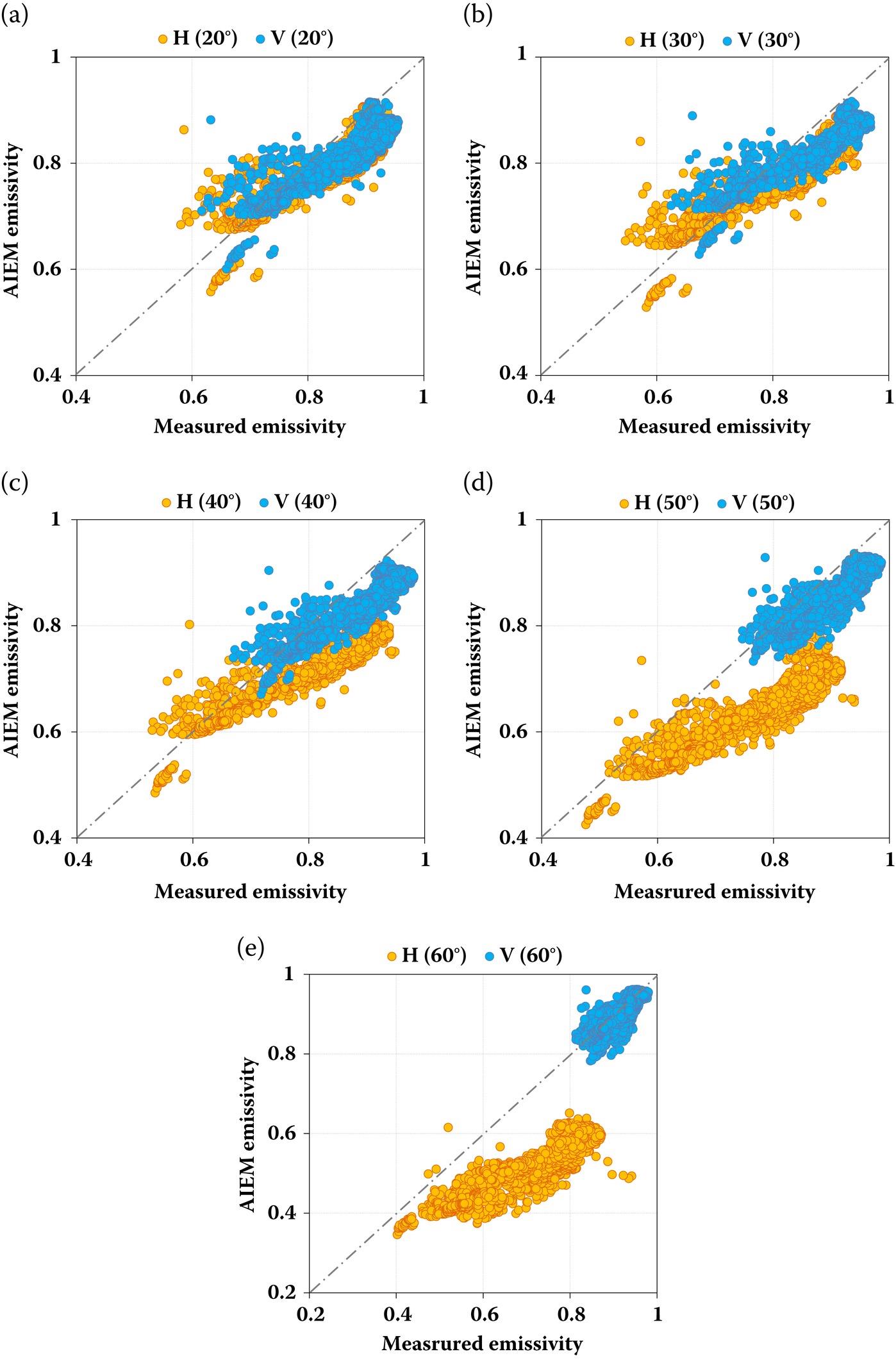
FIGURE 7.8 Comparison of H and V polarized emissivity between AIEM and SMOSREX06 measurements for an exponential correlated surface at 1.41 GHz with look angle of (a) 20°, (b) 30°, (c) 40°, (d) 50°, and (f) 60°.
7.2 SURFACE PARAMETER RETRIEVAL
7.2.1 DATA INPUT–OUTPUT AND TRAINING SAMPLES
In this demonstration, two neural network configurations were devised, with three input parameters for backscattering and four input parameters for bistatic scattering (see Table 7.1). The outputs of the network were normalized surface roughness (RMS height , correlation length ), and soil moisture . Three roughness spectra—Gaussian, exponential, and 1.5-power—were all included in the simulation. The inputs are given in Table 7.1.
TABLE 7.1
Input–Output of a Neural Network
Backscattering (Three-Input) | Bistatic Scattering (Three-Input & Four-Input) | ||
Input | Output | Input | Output |
HH, VV, and HV polarized backscattering coefficients of | Normalized surface roughness and soil moisture | HH, HV, VH, and VV polarized bistatic coefficients | Normalized surface roughness and soil moisture |