5 Sensitivity Analysis of Radar Scattering of Rough Surface
Sensitivity analysis (SA) of electromagnetic waves scattering from a randomly rough surface is pivotal in the field of remote sensing of surface geometrical and dielectric parameters [1–3]. It has been routine to retrieve the geophysical parameters of interest, such as soil moisture and roughness, from the radar scattering measurements [4–7], based on analyzing the sensitivity of the scattering behavior and mechanisms. By capturing the scattering patterns, one could effectively avoid undesired parameters, while devising a means to retrieve the parameters of interest. Knowing how the variation in the model outputs attributes to the variation of the inputs is critical [8]. SA can be on local and global scales [9]. Local SA is a gradient-based method that analyzes only one parameter in a model at a time while fixing all of the remaining parameters to their nominal values [9]. Local SA is simple and computationally efficient. However, it fails to quantify the interactions among all the parameters of interest; hence it may not be a good choice for the nonlinear and non-monotonic model [10]. Global SA, on the other hand, considers the mutual coupling among all the parameters within their ranges. That is, all the parameters in a model are simultaneously analyzed in global SA.
Among the global SA methods, the extended Fourier amplitude sensitivity test (EFAST) [11–16] and entropy-based sensitivity analysis (EBSA) [17], are two common methods. EFAST is one of the most popular methods for SA and is applicable to nonlinear and non-monotonic models, and can quantify the parameter interaction effects as well. Besides, to preserve the maximum information content of the parameters of interest, which are transferred from inputs to outputs of the radar response, EBSA has been proposed to characterize the information content in terms of communications theory, to quantitatively and objectively evaluate the information of the sensor configurations, and to evaluate observational uncertainty that is associated with parameter sampling [18]. In this chapter, we will focus on these two methods and show the numerical results.
5.1 EXTENDED FOURIER AMPLITUDE SENSITIVITY TEST (EFAST)
EFAST, a variance-based, is developed based on FAST and Sobol [19]. The FAST method offers a high-efficiency sampling method that is based on a suitably defined search curve. However, it can only calculate the “main effect” contribution of each input parameter to the model outputs. Thus, it cannot identify the parameter interaction effects on the model outputs. The Sobol method is capable of computing higher interaction terms but is computationally expensive since it adopts Monte Carlo sampling. EFAST fuses the strengths of FAST and Sobol to obtain the total and the main sensitivity indices (SIs) of each input parameter.
The EFAST algorithm mainly includes two steps: sampling and SIs computation. The random sample is first generated by converting the multidimensional parameter space into a 1-D space via a transformation function. The sample size Ns is calculated by using the largest sampling frequency and the number of exploring curves Nr as
where M is a interference factor. To ensure a reasonable sampling density, Saltelli et al. [11] suggested some constraints for the parameters: M = 4, and . Once and Nr are fixed, the number of random samples can be determined.
The second step is to calculate the SIs for each model input, which is expressed by two indices: the main sensitivity index (MSI) and the total sensitivity index (TSI), as
where is the estimated conditional variance of the ith factor, is the estimated conditional variance except for the ith factor, and is the variance of output . is the main (or first-order) sensitivity index of the ith factor, which represents the main contributions of each input parameter to the variance of the model output, and is the total (including higher-order effects) sensitivity index of the ith factor, which considers the interactions among parameters. If differs from , interactions between parameters exist. Both and range between 0 and 1; higher and values suggest more important effects of the ith factor on the output. In Equation (5.4), represents the parameter interaction. For a detailed description and mathematical derivations of EFAST, readers are referred to [9], [11].
5.2 ENTROPY-BASED SENSITIVITY ANALYSIS (EBSA)
For a random rough surface, the scattering response varies nonlinearly with the surface conditions. There exists a well-known problem of uniqueness in the radar response measurement, because of the complex wave-target interactions, as well as uncertainty sources, such as the random characteristics of the rough surface and noise-corrupted radar echoes [20, 21]. It has been proven that, for the backscattering from a randomly rough surface, the Shannon entropy is a good indicator of the sensitivity of radar response to surface parameters because it not only reflects the probabilistic distribution of scattering coefficient but also the deviation [18]. In EBSA, more information on scattering behavior can be preserved through evaluating detailed parameter sensitivities, predicting the scattering signal saturation, estimating the advantage of multi-polarization and multi-angle, and identifying less significant variables. It is convinced that EBSA offers richer details of scattering sensitivity. The procedure EBSA composes of three steps: sampling input data, computing Shannon entropy (SE) and performing sensitivity analysis.
- Sampling input data: generating sufficiently ample normally distributed random samples of surface parameters and bistatic configurations. The range of parameters includes surface roughness, dielectric constant, and bistatic configuration.
- Computing Shannon entropy: feeding the generated samples into the AIEM model to compute the scattering coefficients and the corresponding Shannon entropy SE [22]:
where E is an expected value operator, and H is the Hartley’s information content of observation , and P is the probability density function (pdf), which can be estimated by kernel density method with a Gaussian kernel [23].
- Performing sensitivity analysis: tackling the signal changes in the bistatic scattering pattern by a varying one single parameter with the other parameters remaining unchanged to analyze the radar signal sensitivity to the surface roughness, moisture content, and bistatic configuration at both co- and cross-polarizations are examined.
To assess the performance of SE as well as scattering behavior, we may look into some distribution parameters, including skewness , kurtosis , and standard deviation σstd.
where μ is the mean of the random variable x, and σstd measures statistical dispersion, and measures the asymmetry of the data around the sample mean, and indicates how outlier-prone a distribution would be. Thus, and , equal to zero for a normal distribution. If is negative-valued, the data are spread out more to the left of the mean than to the right. Distributions that are more outlier-prone than the normal distribution have a positive value of , with a negative value of indicating distributions that are less outlier-prone than the normal distribution.
5.3 MONOSTATIC VS. BISTATIC SCATTERING PATTERNS
5.3.1 MONOSTATIC SCATTERING PATTERNS
5.3.1.1 Distribution Response of the Backscattering Coefficient
Figure 5.1 is a scattering coefficient distribution with the normal disturbed rough surface parameter as inputs. The exponential correlation function is used at an incident angle of 45°. In the figure, we can see that, for dry and smooth surface, the scattering coefficient remains normal distributed. With increasing surface roughness and moisture content, the behavior of the rough surface scattering is not normally distributed, as is frequently assumed. It is more likely to be Beta distribution, as shown in Figure 5.2. This disagreement can be described by the departures of the and of returns from normal distribution counterparts. Moreover, we can see that, compared to the surface with varying and , the scattering response deviation is more obvious when varying . Finally, it can be observed that the HH-polarized distribution response is generally similar, but not exactly the same, as the VV-polarized ones.
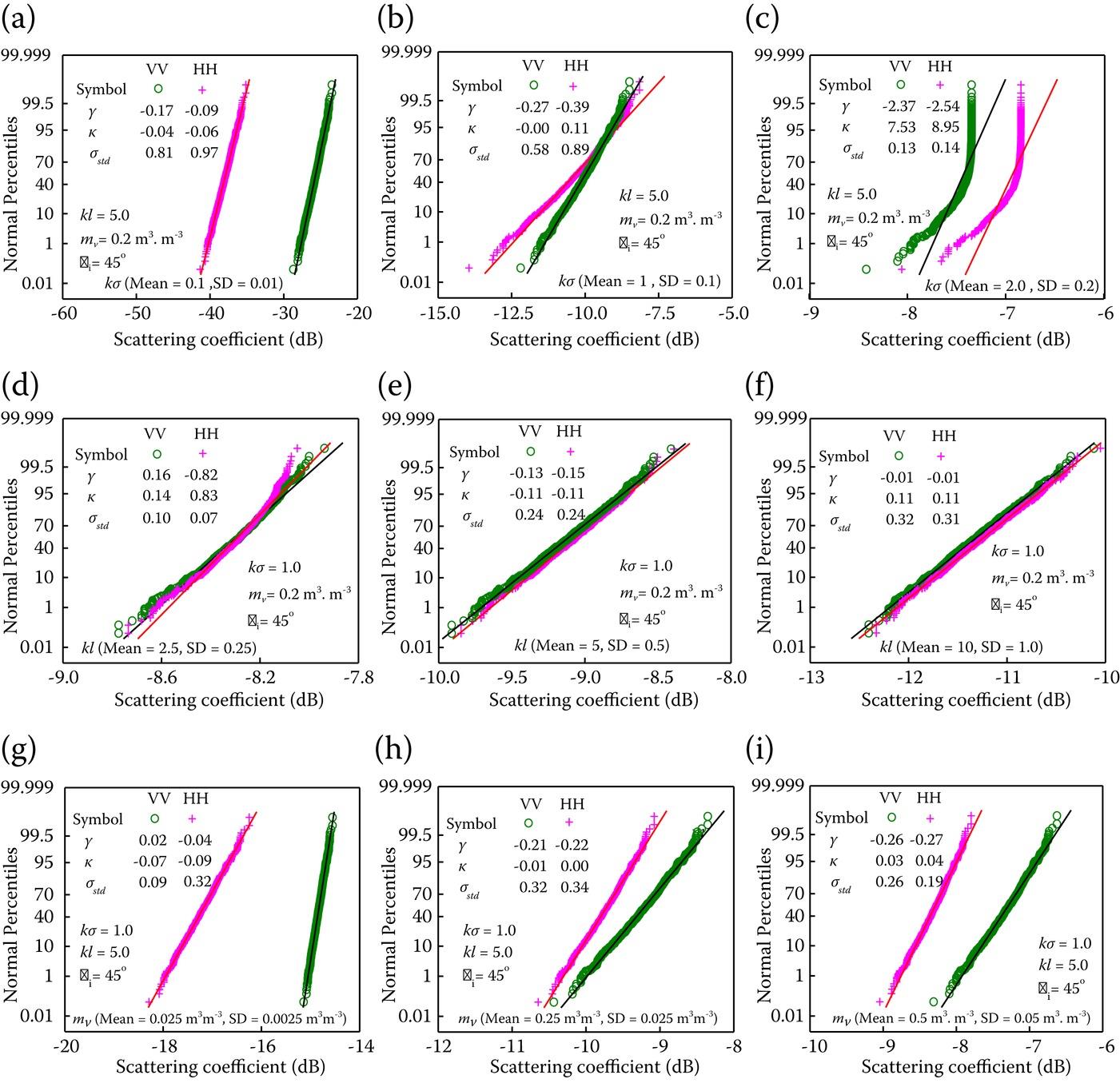
FIGURE 5.1 Probability of the scattering coefficient with normal distributed input surface parameters of (a–c) ; (d–f) ; and (g–i) , at the incident angle of 45°. The solid line is the reference line for a normal distribution fit. (a, d, g) small , , and ; (b, e, h) medium , , and ; and (c, f, i) large , , and . The parameters on upper left of the figures show the distribution of the scattering coefficients, while on the bottom are the input parameters.
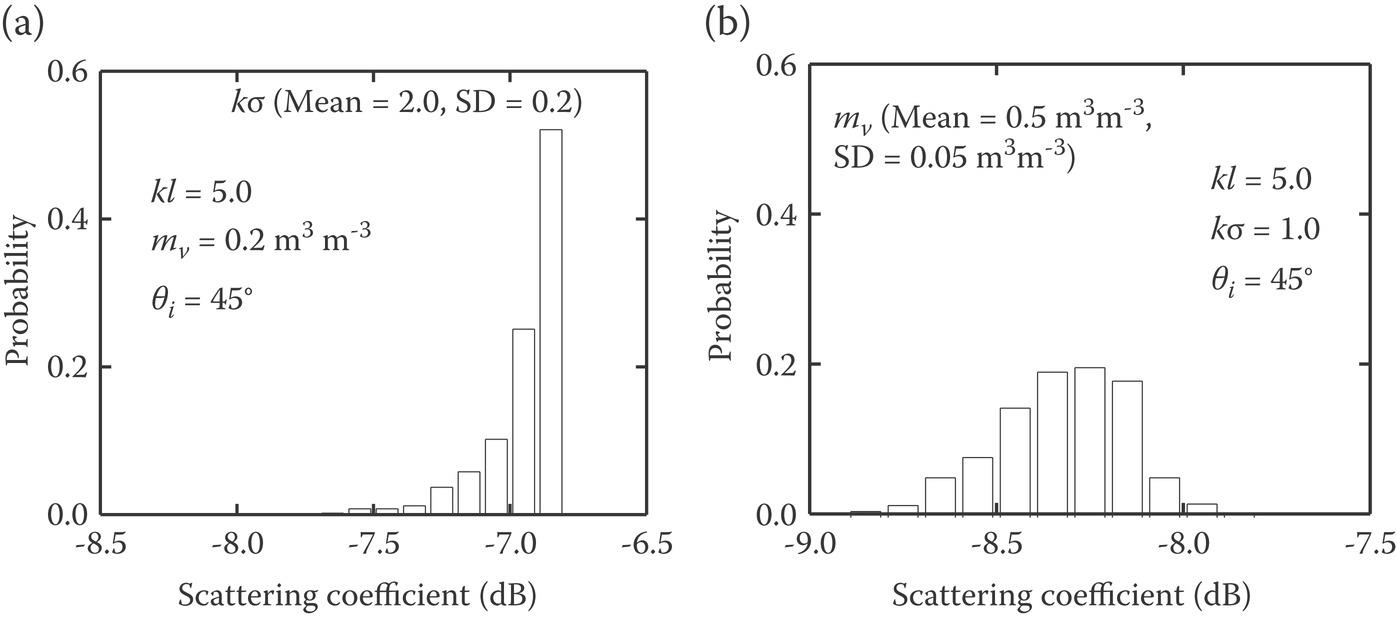
FIGURE 5.2 Probability distribution response of backscattering for rough surface with (a) great roughness; and (b) high water content.
5.3.1.2 SA by Information Entropy
Figure 5.3 is a volumetric slice of SE with three key surface parameters, namely, , , and in the x, y, z directions, respectively. In the figure, we observe that the entropy related to the HH polarization is generally similar to that of VV polarization. Moreover, the backscattering signals were found to be more sensitive to than to and . Those results are highly consistent with those by other methods, such as EFAST methods. The consistency between the different models indicates again the feasibility of the proposed entropy-based method in SA.
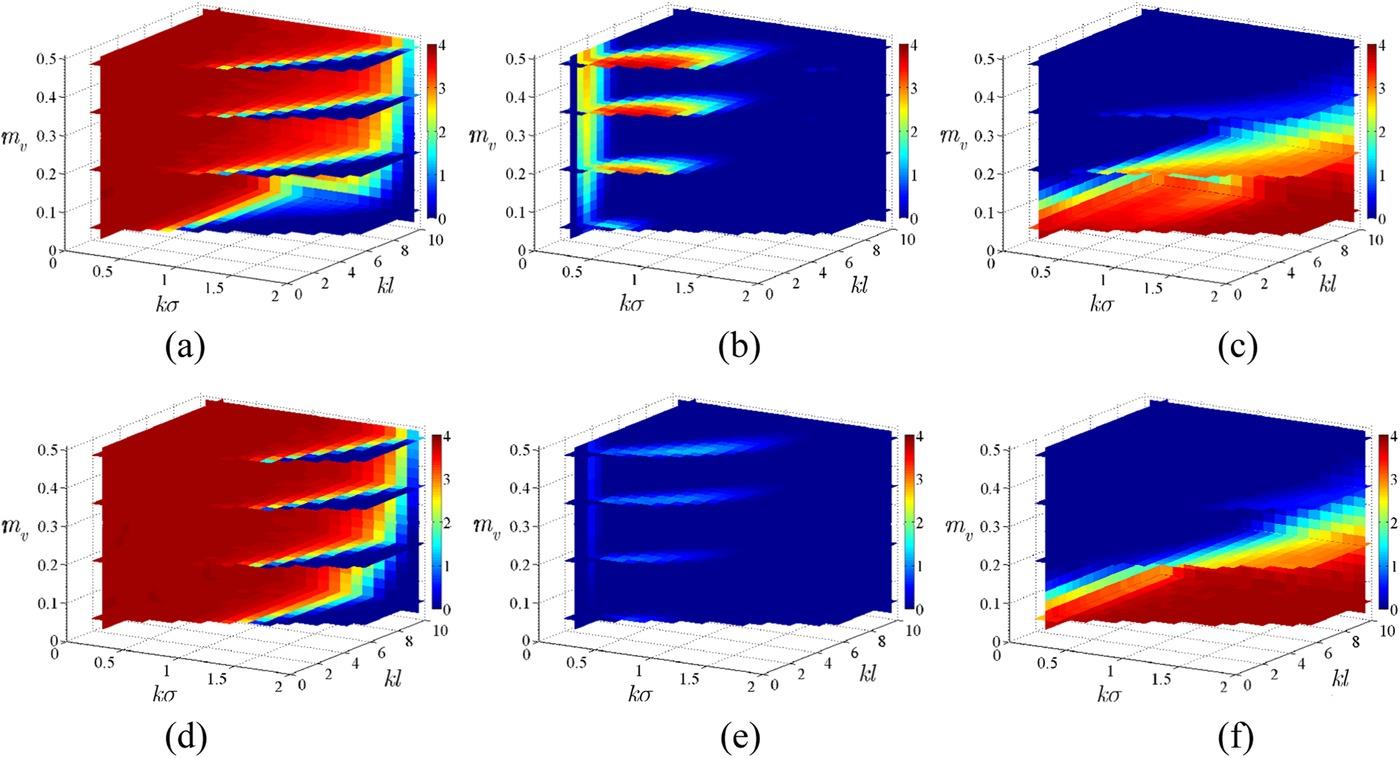
FIGURE 5.3 SE response to rough surface when varying: (a, d) ; (b, e); and (c, f) at a polarization of: (a–c) VV; and (d–f) HH. The incident angle is 45° and the frequency is 1.26 GHz. Normal distribution disturbances were added to the input parameters, with σ equal to 1% of the maximum of the corresponding surface parameters. As an example, SD is set to 0.02, 0.1, and 0.005 when varying: (a, d) ; (b, e) ; and (c, f) , respectively.
Note that, compared to other SA methods, the entropy-based method provide more specific and detailed parameter sensitivity under various rough surface conditions, while there is always only single SA data for global SA or limited data for local SA. Therefore, the proposed method can give us more insight into the scattering behaviors of the rough surface and, thus, ensure better use of microwave scattering data in surface monitoring. Another interesting finding is that it is easy to determine the saturation point of backscattering signals in the form of rapidly decreasing entropy. For example, it can be seen that backscattering signals tend to saturate for a rougher surface, and wet soil prompts the saturation.
5.3.2 BISTATIC SCATTERING PATTERNS
To explore the distribution response of the bistatic scattering coefficient, we start with an intermedium roughness (), with the remaining parameters being fixed to (). Figure 5.4 shows the hemispherical plots of the standard deviation σstd and their values at an incident angle of 50°, for both cross- and co-polarizations. In this plot, the left and right halves correspond to the backscattering and forward scattering direction, respectively, and the horizontal and vertical axes represent the incident and cross planes, respectively. It must be noted that, the exponential correlation function is used because of its good representation of natural surface conditions. Regarding σstd, a stronger co-polarization σstd closes to the specular region, a smaller σstd in the backward region, and the appearance of a minimum HH-polarized σstd around the cross-plane (the vertical axis), the apparent locations of the VV minima along the arc, and a nearly disappearing cross-polarized σstd in the incidence plane (the horizontal axis). Accordingly, a high SE always corresponds to small deviations from a normal distribution and a large standard deviation σstd. Moreover, the overall cross- and co-polarized distribution responses are generally similar, despite not being exactly the same. It is interesting to find that the greatest scattering sensitivity to is found in the backward direction, and the scattering signals always saturate in the forward direction.
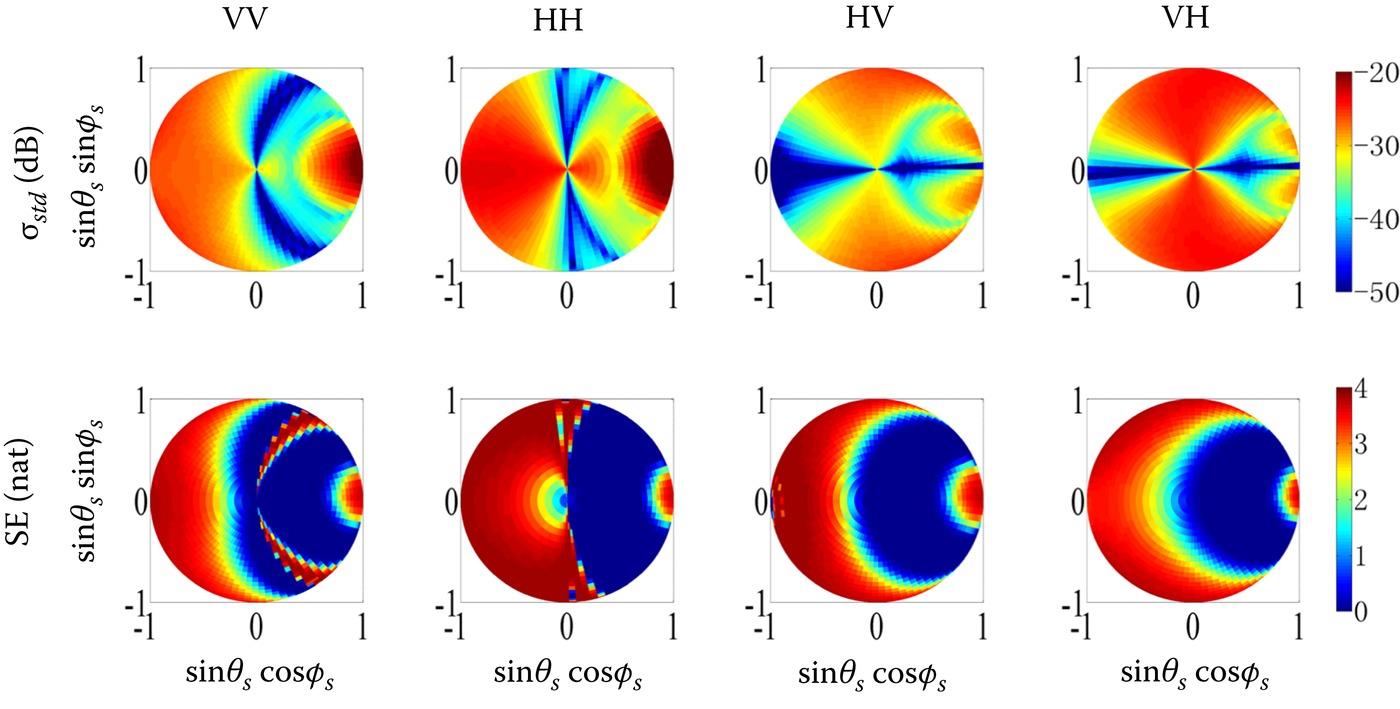
FIGURE 5.4. Hemispherical plots of the standard deviation σstd and the corresponding Shannon entropy () with the normally disturbed as the input: VV (first column); HH (second column); HV (third column); VH (fourth column), at θI = 50°, with kl = 5.0, and .
Figure 5.5 shows the hemispherical plots of the standard deviation σstd, and their values at an incident angle of 50°, for both cross- and co-polarizations. The scattering response to is found to be quite different from that of . A number of significant SE values are found at large scattering angles in the forward and backward directions, for both cross- and co-polarizations, and around the perpendicular planes for co-polarizations. Thus, the effect of correlation length at those directions should be carefully considered in bistatic observation, whereas it has almost no effect on mono-static observation for most rough surface conditions. Moreover, there appears to be a new, nonsensitive scattering response region in the backward direction with small σstd, such as regions at small scattering angles for VV polarization and around the perpendicular scattering plane for HH polarization.
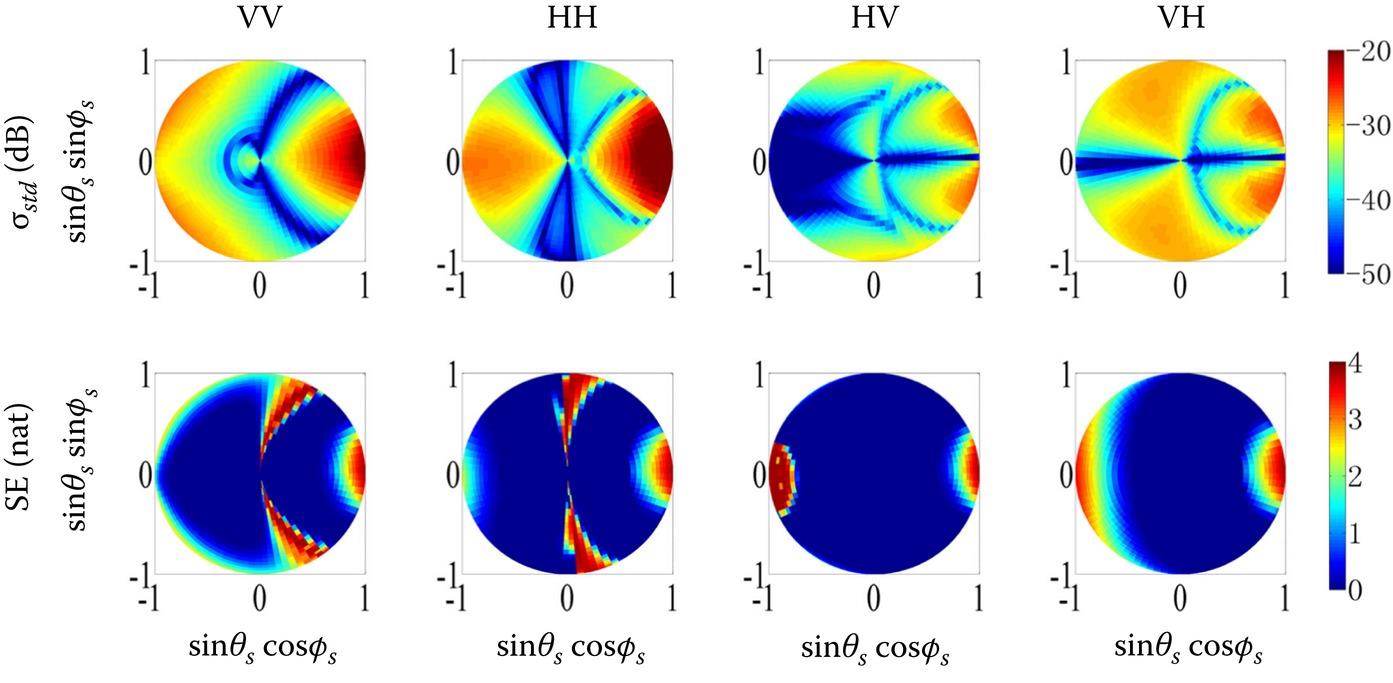
FIGURE 5.5 Hemispherical plots of the standard deviation σstd and the corresponding Shannon entropy () with the normally disturbed as the input: VV (first column); HH (second column); HV (third column); VH (fourth column), at θi = 50°, with , and .
Figure 5.6 shows hemispherical plots of the bistatic scattering coefficient for surface with normally disturbed water content. In general, the scattering sensitivities to are different for all four polarizations. For VV polarization, scattering in the forward direction is more sensitive than in the backward direction. For the HH polarization, the behavior is the opposite.
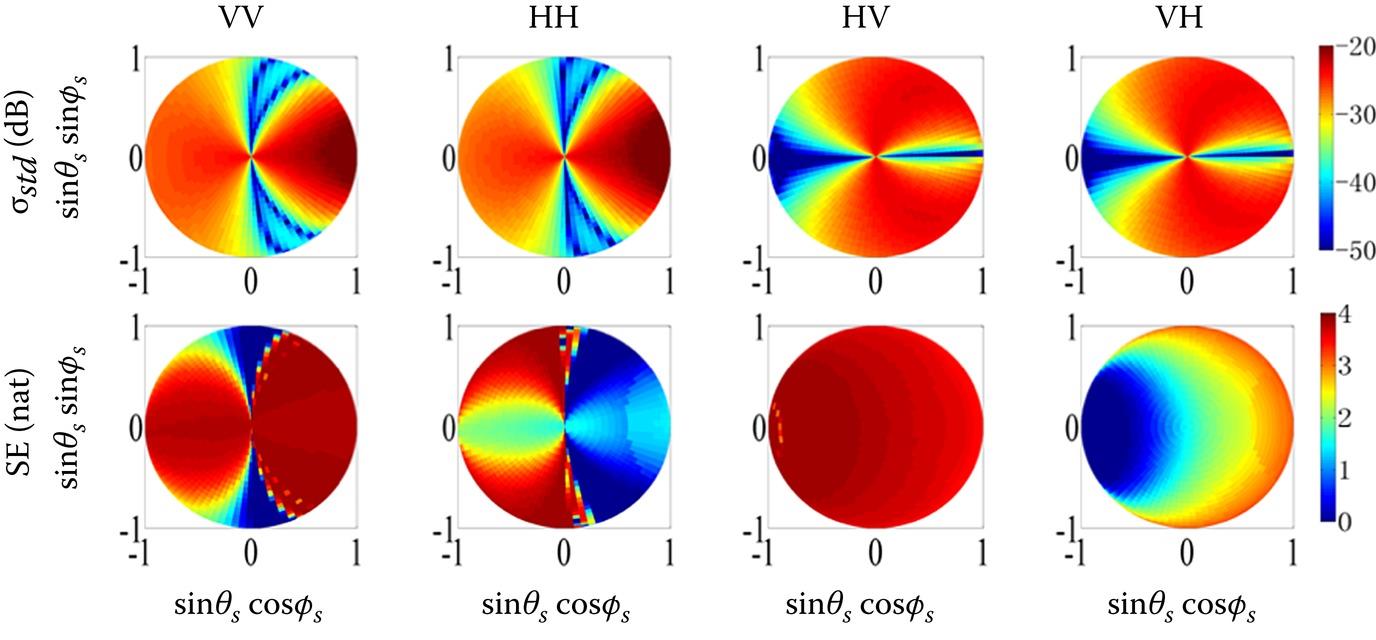
FIGURE 5.6. Hemispherical plots of the standard deviation σstd, and the corresponding Shannon entropy () with the normally disturbed as the input: VV (first column); HH (second column); HV (third column); VH (fourth column), at θi = 50°, with , and .
5.4 DEPENDENCES ON RADAR PARAMETERS
5.4.1 SENSITIVITY TO INCIDENT ANGLE
Figure 5.7 shows the entropy as a function of the incident angles. The SE decreases first and then increases, and the smallest entropy is always found around the small incident angles for a smooth surface, and it shifts toward a greater incident angle for rougher cases. This finding indicates that, for roughness sensing, the backscattering observation at greater incident angles is preferable for a smooth surface, while smaller incident angles for rough surfaces, to retain the maximum information. The distribution of is notably different from that of . The SE curve with varying is monotone, and the scattering signal saturates more quickly for a more wet surface. The above results are based on VV polarization. Similar behavior with HH polarization is observed.
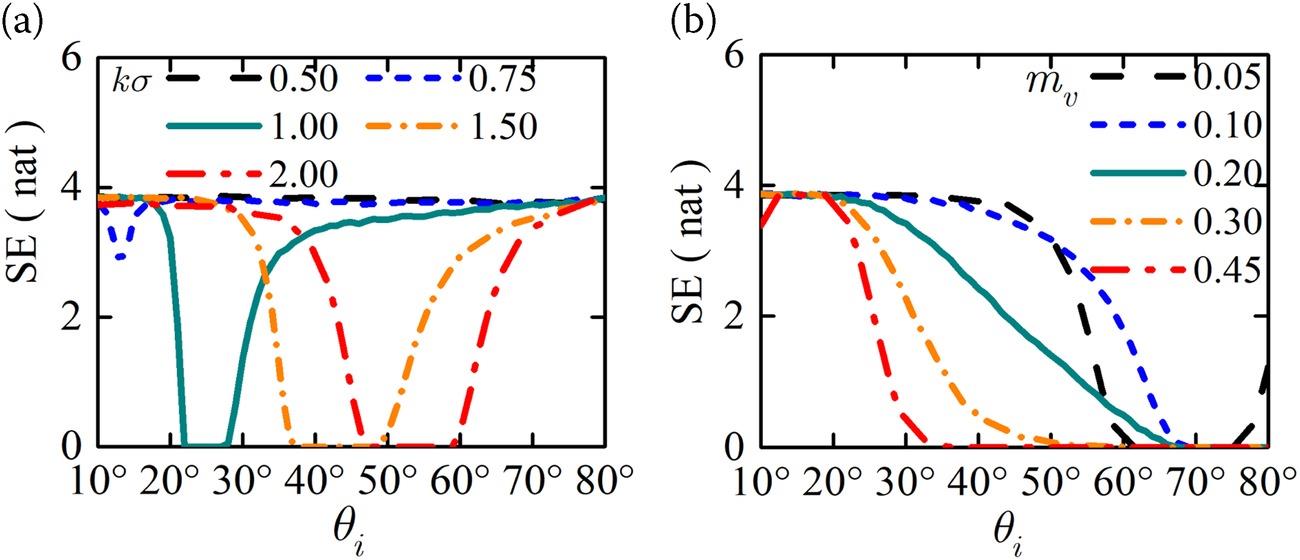
FIGURE 5.7 SE as a function of incident angles for varying (a) normalized rms height (, ) and (b) moisture content ().
5.4.2 SENSITIVITY TO POLARIZATION
Two sets of simulation data with dual-polarization are combined (i.e., the polarization ratio and the polarization difference), as given in Figure 5.8. The figure shows that dual-polarization is expected to provide some improvements in estimating the moisture content compared to single-polarized measurements by reducing the scattering coefficient saturation for rough and wet surfaces, which is a problematic issue in surface parameter retrieving.
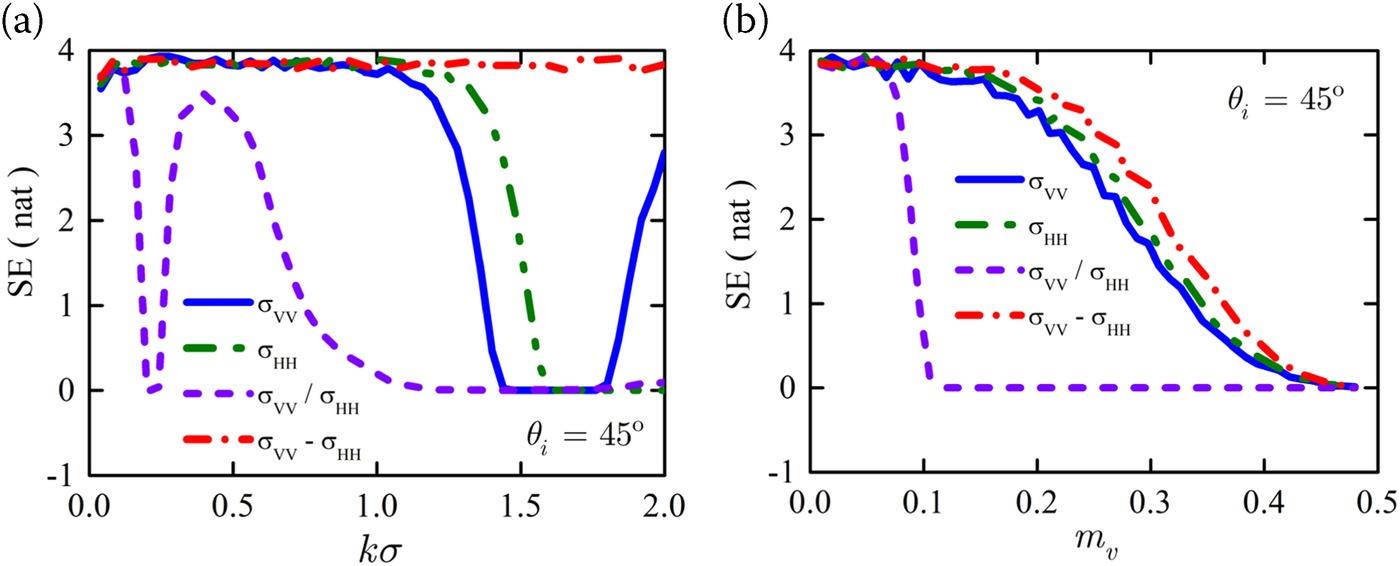
FIGURE 5.8. SE of single- and dual-polarization for varying (a) normalized rms height () and (b) moisture content () at θi = 45°.
5.4.3 SENSITIVITY TO MULTI-ANGLE
For multi-angle observations, we investigate the SE response of two angles (θi = 30°, 45°), and three angles (θi = 30°, 45°, 50°) in simulations, as shown in Figure 5.9. Note that only varying the roughness and moisture content were considered because the scattering response to is comparatively less sensitive. The figure shows that a multi-angle observation can help to address the issue of the scattering signal saturation for wet and rough surfaces, which is similar to the using dual-polarization. It is interesting to note that for multi-angle, which is the combination of backscattering coefficients at two incident angles (σVV(30°)/σVV(45°)), the SE is sensitive to but not to , while the combination of three angles
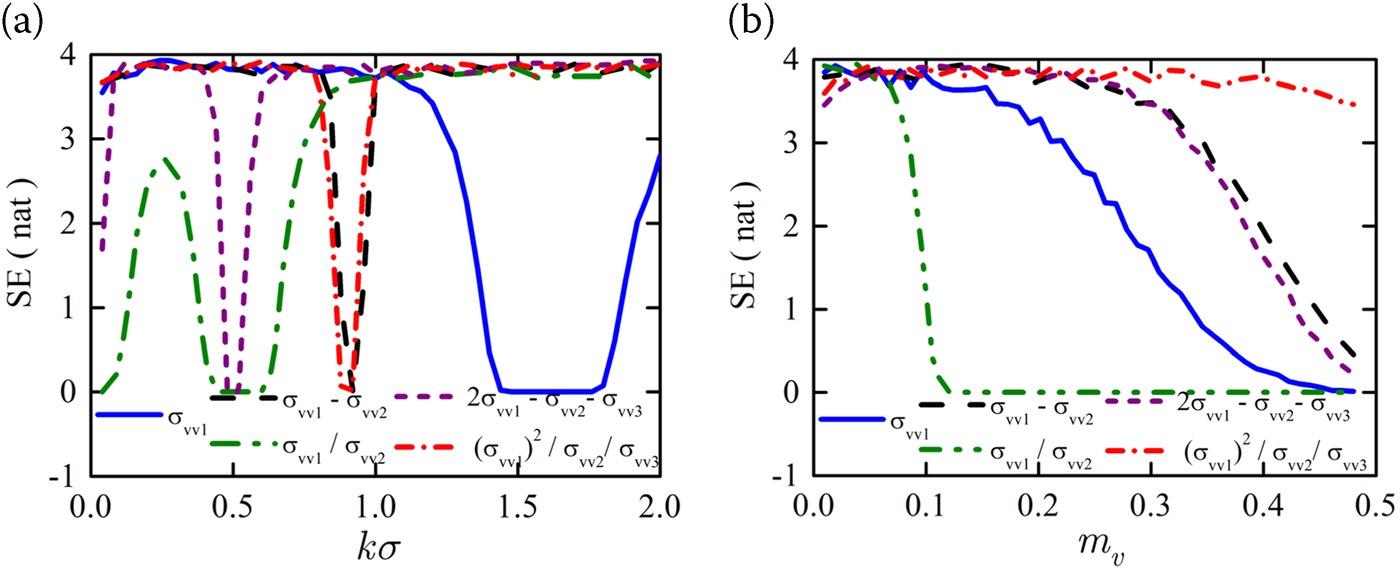
FIGURE 5.9. SE of the single and multi-angle when varying (a) normalized rms height () and (b) moisture content · . σVVi is the VV-polarized scattering coefficient with the incident angle ϑi = 30°, 45°, 50°.
(σVV(30°))2/(σVV(45°)σVV(50°)) shows sensitivity to both and . This finding implies that one can use the ratio between the values of the backscatter data at two angles to estimate the roughness while assigning the soil moisture at a constant value. The soil moisture can then be estimated based on the ratio of the three backscatter values using the estimated roughness. Note that there is an obvious SE dip for a multi-angle observation due to the almost equal scattering coefficients of those angles.
To indicate quantitatively the effect of the angular combination, we compared the sensitivity of bistatic scattering observation, including single input–single output (SISO) and single input–multiple output (SIMO), with a comparison of the mono-static observation in Figure 5.10, which shows the effect of angular combination of bistatic observation on sensitivity to and , with a comparison of mono-static observation for VV polarization. The parameters are given in Table 5.1. The results show that the angular combination can greatly improve the information of interest parameters of the rough surface.
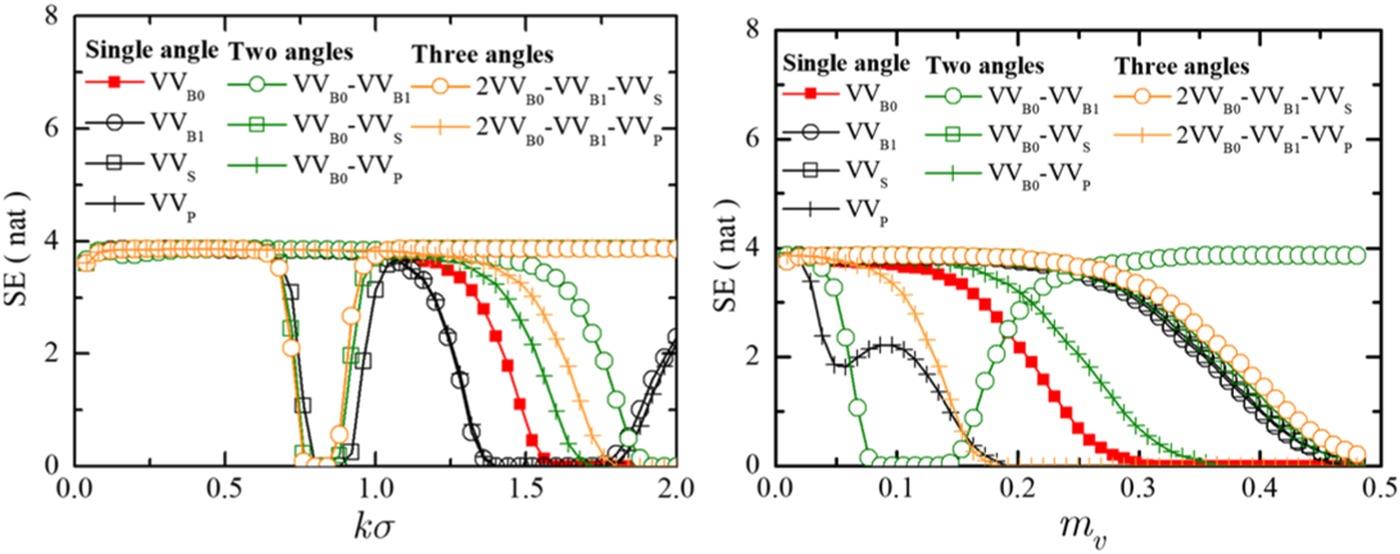
FIGURE 5.10. SE as a function of (a) , with and and (b) , with and ·VVn, n = B0, B1, S, P, B0 and B1 are backscattering observations with (ϑs, ϕs) equal to (50°, 180°) and (40°, 180°), respectively, S shows the observation in the specular scattering plane (50°, 0°), and P in perpendicular plane (50°, 90°) at θI = 50°.
Table 5.1 Angular Combination of Bistatic Observations
Type | Receiving Direction | Receiving Angle | ||
mono-static | SISO | Backward | (50°, 180°) | B0 |
SISO | Backward | (40°, 180°) | B1 | |
SISO | Perpendicular | (50°, 90°) | P | |
SISO | Specular | (50°, 0°) | S | |
SIMO | Backward | (50°, 180°), (40°, 180°) | B0, B1 | |
Bistatic | SIMO | Backward, Perpendicular | (50°, 180°), (50°, 90°) | B0, P |
SIMO | Backward, Specular | (50°, 180°), (50°, 0°) | B0, S | |
SIMO | Backward, Perpendicular | (50°, 180°), (40°, 180°), (50°, 90°) | B0, B1, P | |
SIMO | Backward, Specular | (50°, 180°), (40°, 180°), (50°, 0°) | B0, B1, S |
Note: The incident angles are (50°, 0°). SISO means single input and single output observation; SIMO single input and multi-output.